The 10 Guiding Principles Of GMLP Identified By The FDA, HC, And MHRA
By Shilpa Gampa, Freyr Solutions
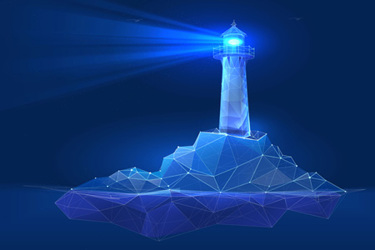
AI/ML-driven medical devices require unique considerations, owing to their complexity as well as the iterative and data-driven nature of their development. In recent years, the complexity of regulatory requirements for AI/ML-integrated medical devices has skyrocketed and has, in turn, posed significant challenges for manufacturers in terms of data security, patient safety, and device quality, hindering their access to global markets.1 The U.S. FDA, Health Canada (HC), and the U.K.’s Medicines and Healthcare Regulatory Agency (MHRA) have come together and identified 10 guiding principles, with the aim of developing good machine learning practices (GMLP) that will address the unique nature of AI/ML-driven devices, in particular:
- Adopt good practices that have been proven in other sectors.
- Tailor practices from other sectors so that they are applicable to medical technology and the healthcare sector.
- Create new practices specific to medical technology and the healthcare sector.
The guiding principles also identify areas where the International Medical Device Regulators Forum (IMDRF), International Organization for Standardization (ISO), and other collaborative bodies could work to advance GMLP for medical device development.2,3 This editorial will delve into the details of the 10 guiding principles released by the FDA (based on collaborative work with HC and MHRA) in October 2021.
1. Leverage Multidisciplinary Expertise throughout the Product Life Cycle
It is crucial to have multidisciplinary expertise at every stage of medical device development, from the initial ideation to the development process, throughout the device life cycle, including post-deployment. The experts involved in AI/ML medical device development must have an in-depth knowledge and understanding of the device’s intended use, clinical workflow, benefits, and potential risks to patient safety. Since AI/ML is mostly developed by technology engineers with knowledge gaps in regulatory requirements for medical device development, this rule stresses the importance of having a team of experts with multidisciplinary expertise to ensure that ML-enabled medical devices are safe and effective and address clinically meaningful needs over their life cycle. It also ensures compliance with medical device development in line with the QMS, consensus standards, and applicable regulatory requirements.2,3
2. Implement Good Software and Engineering Practices for AI/ML-based Medical Devices
Data security, integrity, and privacy are of utmost importance throughout the product life cycle of AI/ML devices. It is strongly recommended that manufacturers protect their devices against unauthorized access, privilege escalation, and data exfiltration. Therefore, they must implement the fundamentals of good software engineering practices, data quality assurance, data management, and robust cybersecurity practices from the initial stages. Such practices include methodical risk management and design processes that can appropriately capture and communicate design, implementation, and risk management decisions and rationales, while also ensuring data authenticity and integrity. For example, during the risk management process, the manufacturer shall assess and mitigate risks that occur specifically to ML libraries, chosen software architecture, data processing, design transfer, specific selection of data, unforeseen operation conditions, etc.2,3
3. Represent the Intended Patient Population through Clinical Study Participants and Data Sets
In clinical study and training and test data sets, manufacturers must sufficiently represent the relevant characteristics of the intended patient population (e.g., age, gender, sex, race, and ethnicity), use, and measurement inputs in a sample of adequate size. This will ensure that the results are aligned with the population of interest. They must also manage any bias, promote appropriate and generalizable performance across the intended patient population, assess usability, and identify circumstances where the model may underperform. The potential list of biases might include:
- nonrepresentative patient populations, e.g., volunteers, sex, race, age, size, weight, diseases, treatments, social and geographic environment;
- data collection (e.g., types of questionnaires) or using channels (e.g., social media) that are used predominantly by certain groups;
- attributes that are irrelevant for the expected output;
- confusion of correlation and causation;
- preparation of source data (e.g., histopathological slides);
- specific data sources (e.g., different types, accuracy);
- location of data collection (e.g., size and type of hospital, rural versus urban);
- aggregation that combines data that are not representative of the single population; and
- “over-curation” (e.g., excluding data from poor-quality MRI scans that, however, are common). “Over-curation” may also exclude certain patient profiles; further, the manufacturer should specify the distribution of input data that is representative of the target system/population. Characteristics can include demographics such as age, sex, race, health status, comorbidities, social status, education, and motivation to participate in studies.2,3
NOTE: Even if all individual data sets meet the specification, the distribution of data might not be representative and/or may cause a bias.
4. Maintain Training Data Sets as Independent from Test Data Sets
You need to select and maintain training and test data sets in a way such that they are independent of one another. You should consider and address all potential sources of dependence, including patient details, data acquisition, and site factors. Test data refers to the subset of the data set and not the part of the training data set that is used to evaluate the ML model accuracy after its primary vetting by the validation data set. Quality tests are performed to minimize the noise and variance of the test data in order to maximize the performance accuracy of the ML algorithm applied to it.2,3 Also, the manufacturer should validate that the test and training data meet the specified criteria.
Descriptive statistics may include the following:
- Calculation of distributions (histograms)
- Mean/average values
- Quartiles
- Joint distribution of features, correlation, etc.
Label leakage examples include:
- In the sorting (e.g., first, the data of healthy persons, then of ill persons)
- In the hospital (e.g., if the severe cases originate from just one institution)
- In images (e.g., for skin cancer, one must always see a ruler)
5. Use the Best Available Methods for Selected Reference Data Sets
You need to develop the AI/ML device model with a relevant and robust reference data set (a reference standard) that consists of well-characterized and clinically relevant data for the medical device’s end use. If reference data sets are available, you should use them in model development, evaluation, and testing, which will, in turn, help you promote model robustness and generalizability among the intended patient population. The risk analysis examines the consequences of wrong reference data (e.g., wrong gold standard, wrong comparison).2,3
6. Tailor the Model Design to the Available Data and Ensure that it Reflects the Device’s Intended Use
To support the active mitigation of known risks, such as overfitting, performance degradation, and security risks, you must tailor the model design to suit the available data. It is helpful if you possess a thorough understanding of the clinical benefits and risks related to the device, and you can use the model to derive clinically meaningful performance goals for testing. Additionally, the model should support the device safely and effectively in achieving its intended use. Considerations include the impact of both the global and local performance of the device, uncertainty or variability in the device’s inputs and outputs, intended patient populations, and clinical use conditions.2,3
7. Focus on the Performance of the Human–AI Team
Where the model has a “human in the loop,” address considerations of human factors and the human interpretability of the model outputs. Moreover, place added emphasis on the performance of the human–AI team rather than just on the performance of the model in isolation. It is important for the manufacturer to identify all the risks related to the usability of the device during their risk analysis and evaluate all the safety-relevant use scenarios. Usually, the summative evaluation of the usability evaluation covers all the safety-relevant use scenarios and evaluates the effectiveness over all the risk mitigation measures.2,3
8. Use Testing to Demonstrate the Device’s Performance during Clinically Relevant Conditions
This guiding principle focuses on the requirement to generate clinically relevant, statistically significant information/data on a device’s performance that is independent of the training data set. The AI model selection, AI model evaluation metrics, optimization parameters, and testing play a crucial role in obtaining adequate and compliant data required to gain regulatory approvals. You should also consider including the intended patient population, important subgroups, clinical environment, use by the human-AI team, measurement inputs, and potential confounding factors in generating the use test data. It is recommended that you consider conducting early interactions with the appropriate agency through Q-sub meetings/Pre-sub meetings to gather the agency’s feedback on all the different considerations that apply to the use testing data of the subject device.2,3
9. Provide Users with Clear, Essential Information
You must provide clear and contextually relevant information to the intended users (like healthcare providers or patients). The relevant user information includes:
- The product’s intended use and indications for use
- Performance of the model for appropriate subgroups
- Characteristics of the data used to train and test the model
- Acceptable inputs
- Known limitations
- User interface interpretation
- Clinical workflow integration of the model
Additionally, you need to make users aware of device modifications and updates from real-world performance monitoring (when the basis for decision-making is available) and provide them with a means to communicate product concerns to the developer.2,3
10. Monitor Deployed Models for Performance and Manage Re-training Risks
With respect to safety and performance improvement, deployed models can be monitored during their “real world” use. After deployment, when models used by the human–AI team are periodically or continually trained, you can put appropriate post-deployment controls in place, including a post-market surveillance (PMS) plan, an incident response plan, and version and configuration controls, to manage risks of overfitting, unintended bias, or degradation (e.g., data set drift) that may impact safety and performance. Also, it is recommended that manufacturers assess the design changes based on the regulatory requirements before implementing them.2,3
Conclusion
Rapid innovations and developing technologies pose enormous challenges for makers of AI- and ML-driven devices when it comes to aligning with patients’ needs and stringent regulatory requirements. Maintaining sole focus on such devices’ quality, security, and safety aspects during their development is the key to a successful market entry. Failure to meet these requirements will lead to device recalls and hefty fines from regulatory agencies. Thus, device manufacturers incorporating AI/ML into their devices must adhere to the relevant government laws, regulations, and GMLP to ensure a compliant market entry.4,5,6
References
- “Good Practices for Health Applications of Machine Learning: Considerations for Manufacturers and Regulators.” ITU Publications. September 2022. Available at https://www.itu.int/dms_pub/itu-t/opb/fg/T-FG-AI4H-2022-2-PDF-E.pdf.
- “Good Machine Learning Practice for Medical Device Development: Guiding Principles.” U.S. FDA. October 27, 2021. Available at https://www.fda.gov/medical-devices/software-medical-device-samd/good-machine-learning-practice-medical-device-development-guiding-principles.
- “Good Machine Learning Practice for Medical Device Development: Guiding Principles.” GOV.UK. October 27, 2021. Available at https://www.gov.uk/government/publications/good-machine-learning-practice-for-medical-device-development-guiding-principles/good-machine-learning-practice-for-medical-device-development-guiding-principles.
- Deshpande, R. “Machine Learning for Medical Devices: Best Practice Guideline.” Scilife. March 17, 2023. Available at https://www.scilife.io/blog/machine-learning-medical-devices-guidelines.
- Modic, E.E. “4 Key Challenges in Sustaining Compliance in the Medical Device Industry.” Today’s Medical Developments. June 17, 2022. Available at https://www.todaysmedicaldevelopments.com/news/4-key-challenges-medical-devices-medtech/
- Valentine, L., and C. Nelson. “Good Machine Learning Practice: Guiding Principles.” Gliff. March 24, 2022. Available at https://gliff.ai/insights/articles/what-the-principles-for-good-machine-learning-practice-mean/.
About The Author:
Shilpa Gampa is a regulatory affairs strategist at Freyr Solutions with expertise in FDA submissions and C-level executive consultation for developing go-to-market strategies. She is acting as the regional delivery head for America’s clients at Freyr, in her primary role for global market submissions. She also leads the Central Consulting Services at Freyr. Gampa has extensive experience in regulatory strategies for various regulated medical products, including combination products, medical devices, IVDs, and digital health devices.