A Look At AI-Driven Medtech For Rare Disease Diagnosis
By Amber Freed, Jacob Tiller, and Sam Patel, SLC6A1 Connect
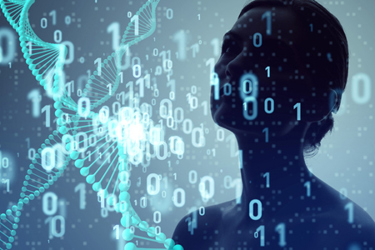
Rare diseases affect over 300 million people worldwide, with more than 7,000 identified conditions.1 Despite this prevalence, patients often face a diagnostic odyssey lasting 5 to 7 years, leading to multiple misdiagnoses and unnecessary treatments.2 This delay exacerbates disease progression and limits access to targeted therapies and clinical trials.3
Recent advances in artificial intelligence (AI), including machine learning (ML), natural language processing (NLP), and deep learning models, are transforming rare disease diagnosis and treatment. AI-powered genome analysis, facial recognition software, and symptom-matching algorithms are accelerating diagnosis, improving accuracy, and expanding treatment options.4,5
This article explores how AI is revolutionizing rare disease detection and management, highlighting key innovations and case studies.
The Diagnostic Challenge In Rare Diseases
Rare diseases pose significant diagnostic challenges. Many conditions lack clear diagnostic criteria, and symptoms often overlap with more common diseases, leading to frequent misdiagnoses.6 A shortage of specialists, limited clinician awareness, and fragmented diagnostic pathways further contribute to delayed diagnoses.7
The consequences of delayed diagnosis include:
- Disease progression: Missed early intervention opportunities
- Limited access to therapies: Patients may be excluded from experimental treatments and clinical trials8
- Increased healthcare costs: Unnecessary tests and treatments add financial strain9
AI-driven diagnostics offer a powerful solution to accelerate accurate diagnoses, reduce costs, and improve patient outcomes.10
AI-Assisted Symptom Recognition And Pattern Matching
AI-assisted symptom recognition and pattern matching leverages machine learning to analyze vast amounts of medical data, including patient histories, clinical notes, and research publications, to identify complex symptom patterns indicative of rare diseases. These algorithms, often employing deep learning techniques, can detect subtle correlations and connections between seemingly disparate symptoms that might be missed by human clinicians. By training on extensive datasets, AI models learn to recognize the characteristic signatures of rare conditions, even when those conditions are exceptionally rare or present with atypical symptoms. This capability significantly enhances diagnostic accuracy and accelerates the recognition of ultra-rare diseases, reducing the time patients spend searching for answers.11
- FindZebra: Acts as an AI-powered search engine specifically designed to aid physicians in diagnosing rare conditions. It uses natural language processing to understand clinical descriptions provided by physicians, effectively translating their input into a format the AI can analyze. FindZebra then searches its extensive database of medical literature and rare disease information, using AI algorithms to match the patient's described symptoms with potential diagnoses. The system presents physicians with a ranked list of possible rare conditions, along with supporting evidence from medical literature, helping them explore diagnostic possibilities they might not have otherwise considered. This tool empowers physicians with rapid access to a wealth of information, streamlining the diagnostic process and potentially uncovering rare conditions earlier.12
- Undiagnosed Diseases Network (UDN): Uses AI-driven tools to compare individual patient cases with a global database of rare diseases. By pooling data from numerous patients and institutions, the UDN's AI algorithms can identify similarities between cases, even when individual presentations are complex or incomplete. The AI analyzes patient symptoms, medical history, and genetic information, comparing it to aggregated data to find potential matches and identify patterns that might suggest a specific rare disease. This collaborative, AI-powered approach enhances diagnostic efficiency by connecting patients with similar cases worldwide, accelerating the process of identifying ultra-rare conditions and fostering the sharing of knowledge among clinicians and researchers.11,13
AI-Driven Facial Phenotyping For Genetic Syndromes
Artificial intelligence has significantly advanced the field of medical genetics, particularly through AI-driven facial phenotyping tools that aid in the diagnosis of genetic syndromes. These tools analyze facial features to detect patterns associated with various genetic conditions, enhancing diagnostic accuracy and efficiency.
- Face2Gene – A widely used AI-powered application that helps clinicians evaluate patients for genetic syndromes by analyzing facial features. It employs deep learning algorithms to compare patient photos with a vast database of images associated with known genetic disorders, providing a list of potential diagnoses ranked by relevance. Studies show it can suggest correct diagnoses within the top 10 results in most cases.¹⁴
- DeepGestalt – Developed as part of the Face2Gene suite, DeepGestalt uses deep convolutional neural networks to identify facial features indicative of genetic syndromes. Trained on over 26,000 patient images, it can recognize patterns corresponding to more than 215 genetic conditions with a top 10 accuracy of 91%. In comparative studies, DeepGestalt has outperformed clinical experts in identifying certain syndromes, highlighting its potential as a supportive tool in genetic diagnostics.¹⁵
- GestaltMatcher – An AI-driven platform that enhances the diagnosis of ultra-rare genetic disorders by comparing patient facial images to a global database. It creates a multidimensional representation of facial features, allowing for the identification of similarities even in conditions not previously documented. This approach facilitates the recognition of novel syndromes and supports clinicians in diagnosing atypical presentations of known disorders.¹⁶
- GestaltMML – An evolution in AI-driven diagnostics, GestaltMML integrates facial images with clinical data and demographic information through a multimodal machine learning approach. This comprehensive analysis improves prediction accuracy for a wide range of genetic diseases, effectively narrowing down possible diagnoses in complex cases.¹⁷
- PhenoScore – Developed at Radboud University Medical Center, PhenoScore combines facial analysis with other clinical data to improve diagnostic accuracy. It uses a 3D imaging system to capture detailed facial structures, which are then analyzed using AI algorithms. PhenoScore enhances diagnostic precision by integrating facial morphology with clinical symptoms, making it particularly useful in complex genetic cases.¹⁸
- Cliniface – An open-source tool that uses 3D imaging and AI to analyze facial morphology. Unlike traditional 2D imaging techniques, Cliniface can identify subtle dysmorphic features that may not be apparent in standard clinical assessments.¹⁹
- AI-Powered Wearable Devices – Emerging wearable technologies, such as smart glasses equipped with AI, are beginning to play a role in real-time facial analysis during patient consultations. These devices capture and analyze facial features instantly, providing immediate diagnostic insights. AI-integrated wearables have the potential to assist clinicians in remote or resource-limited settings by facilitating faster and more accessible assessments of genetic syndromes.²⁰
Regulatory Approval And Compliance
AI-driven diagnostic tools must adhere to stringent regulatory guidelines to ensure their safety and clinical efficacy. In the United States, the Food and Drug Administration (FDA) and, in Europe, the European Medicines Agency (EMA) require rigorous validation of AI models before approval.19 This process includes extensive testing, clinical studies, and performance evaluations to assess algorithm accuracy, sensitivity, and specificity. Given the evolving nature of AI, regulatory frameworks must adapt to challenges such as algorithmic bias and the continuous learning capabilities of AI models.20 Establishing clear approval pathways not only safeguards patient safety but also fosters innovation by ensuring AI-driven diagnostics meet the same high standards as other medical technologies.21
The Future of AI in Rare Disease Diagnostics
AI is poised to bridge diagnostic gaps and enable precision medicine for rare diseases by enhancing early detection, increasing diagnostic speed, and improving accuracy. By integrating machine learning, natural language processing, and advanced imaging, AI is enabling earlier detection, improving diagnostic accuracy, and expanding access to specialized expertise. Tools like AI-assisted symptom recognition, facial phenotyping, and genomic analysis are reducing diagnostic delays, providing clinicians with powerful, data-driven insights that enhance decision-making. Multi-modal AI, which combines clinical, genetic, and imaging data, is further refining diagnostic precision, particularly for ultra-rare conditions with limited case studies. As AI continues to evolve, its integration into clinical workflows will be essential, supporting physicians with real-time diagnostic insights and improving early screening efforts, especially in underserved regions. With continued advancements and ethical oversight, AI will pave the way for groundbreaking innovations in rare disease diagnostics, ultimately improving patient care and expanding access to life-saving treatments.
References
- Nguengang Wakap S, Lambert DM, Olry A, et al. Estimating cumulative point prevalence of rare diseases: analysis of the Orphanet database. Eur J Hum Genet. 2020;28(2):165–173.
- National Organization for Rare Disorders (NORD). Rare Disease Facts and Statistics. 2018.
- Boycott KM, Rath A, Chong JX, et al. International cooperation to enable the diagnosis of all rare genetic diseases. Am J Hum Genet. 2019;105(3):389–397.
- Gurovich Y, Hanani Y, Bar O, et al. Identifying facial phenotypes of genetic disorders using deep learning. Nat Med. 2019;25(1):60–64.
- Jaganathan K, Kyriazopoulou Panagiotopoulou S, McRae JF, et al. Predicting splicing from primary sequence with deep learning. Cell. 2019;176(3):535–548.e24.
- Chong JX, Buckingham KJ, Jhangiani SN, et al. The genetic basis of Mendelian phenotypes: discoveries, challenges, and opportunities. Am J Hum Genet. 2015;97(2):199–215.
- Lynch SA, Monaghan KG, Edwards JG. Ethical and practical challenges in the clinical application of next-generation sequencing: a resource for clinicians. Am J Med Genet A. 2017;173(7):174–189.
- Taruscio D, Floridia G, Groft SC, et al. Advancing rare diseases research: the need for an international rare diseases registry platform. Orphanet J Rare Dis. 2020;15(1):207.
- Wishart DS. Metabolomics in drug discovery and development: a potential tool for precision medicine. Clinical Chemistry.
- Rieke N, Hancox J, Li W, et al. The future of digital health with federated learning. NPJ Digit Med. 2020;3:119.
- Dragusin R, Petcu P, Lioma C, et al. FindZebra: a search engine for rare diseases. Int J Med Inform. 2013;82(6):528–538.
- Gahl WA, Markello TC, Toro C, et al. The National Institutes of Health Undiagnosed Diseases Program: insights into rare diseases. Genet Med. 2012;14(1):51–59.
- Hsieh T-C, Bar-Haim A, Moosa S, Ehmke N, Gripp KW, Pantel JT, et al. GestaltMatcher facilitates rare disease matching using facial phenotype descriptors. Nature Genetics. 2022;54(3):349–357.
- Marwaha A, Chitayat D, Meyn MS, Mendoza-Londono R, Chad L. The point-of-care use of a facial phenotyping tool in the genetics clinic: Enhancing diagnosis and education with machine learning. 2021 Apr;185(4):1194–1201.
- He J, Baxter SL, Xu J, et al. The practical implementation of artificial intelligence technologies in medicine. Nat Med. 2019;25(1):30–36.
- Topol E. High-performance medicine: the convergence of human and artificial intelligence. Nat Med. 2019;25(1):44–56.
- Martinez-Martin, N. (2019). What Are Important Ethical Implications of Using Facial Recognition Technology in Health Care? AMA Journal of Ethics, 21(2), E180-187.
- van der Donk R, Koolen D, Smeets D, et al. PhenoScore quantifies phenotypic variation for rare genetic diseases by combining facial analysis with other clinical features using a machine-learning framework. Nat Genet. 2023;55(9):1598–1607.
- Palmer, R. L., Helmholz, P., and Baynam, G.: “Cliniface: Phenotypic Visualisation And Analysis Using Non-Rigid Registration Of 3d Facial Images,” Int. Arch. Photogramm. Remote Sens. Spatial Inf. Sci., XLIII-B2-2020, 301–308.
- Paragon Institute. Healthcare AI Regulation: Guidelines for Maintaining Public Safety and Innovation. 2023.
- Hogan Lovells. JPM2025: Regulation of artificial intelligence: Navigating a new frontier in health care. 2024.
About The Authors:
Amber Freed is the founder and CEO of SLC6A1 Connect, a nonprofit dedicated to advancing research and treatments for SLC6A1-related disorders and rare diseases. After her son’s diagnosis with the ultra-rare condition, she became a leading advocate, driving scientific collaborations, securing funding, and accelerating drug development. Freed works closely with researchers, industry leaders, and policymakers to push for innovative solutions in rare disease therapeutics.
Jacob Tiller is the chief scientific officer of SLC6A1 Connect. In this role, he contributes to publications and develops strategies for increasing public support of scientific efforts. He joined the organization in 2021 and has worked closely with leading scientists and physicians with the goal of improving patient outcomes for those with the SLC6A1 mutation. Tiller has served in a number of research roles with focuses ranging from gene therapy to EEG analysis and signal processing. He is pursuing a degree in biophysics on the pre-medical track at Brown University and aspires to become a physician himself to directly aid those impacted by rare diseases.
Sam Patel, Ph.D., is a scientist and strategist specializing in rare disease research, precision medicine, and bioethics, with expertise in iPSC disease modeling, CRISPR/Cas9, and high-throughput screening. They have led initiatives in building research programs, establishing laboratories, and managing multidisciplinary teams to advance translational research. Passionate about science communication and patient advocacy, they bridge the gap between bench science and strategic leadership, driving innovation in rare neuromuscular and neurodegenerative disorders. Patel holds a Ph.D. in biomedical sciences. Connect on LinkedIn.