Adaptive AI-Driven Medical Devices In The US: Regulatory Guidelines
By Jessica Chen and Thuha Tran, San Jose University
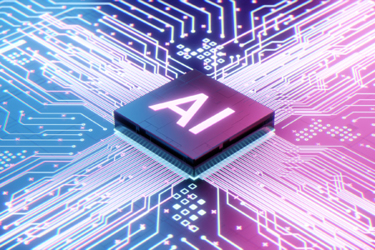
AI-assisted medical devices have actually existed for some time in the healthcare industry, predominantly in the fields of radiology, cardiology, and general practice/internal medicine.1 Computer-aided detection (CAD) software from Paige Prostate2 is already FDA-approved and set to receive marketing authorization. However, it does not currently use machine learning algorithms, generally called adaptive AI, which is the source of a lot of buzz lately. Adaptive AI heavily utilizes large language models (LLM) to imitate human rationality and communication, and sometimes in a more efficient way.
In the United States, the FDA has addressed the recent boom in AI technologies. However, there is more to be scrutinized for patient health data, safety, and privacy. Adaptive AI promises a wide array of medical solutions that will certainly revolutionize the efficiency of healthcare moving forward.
In this two-part article series, we delve into the evolving landscape of AI-assisted devices in healthcare, focusing particularly on the emergence of Adaptive AI. In this article, we will discuss the proposed regulatory considerations surrounding these technologies. In part 2, we will discuss ethical concerns with patient health data, safety, and privacy.
What Are Adaptive AI-Driven Medical Devices?
Adaptive AI falls under the category of “software used in medical devices.” Software as a medical device (SaMD), as defined by the International Medical Device Regulators Forum (IMDRF), refers to software intended for medical purposes without being an integral part of hardware medical devices. Examples of SaMDs include imaging, monitoring, and CAD software. Particularly, adaptive AI-driven SaMD products are equipped with algorithms designed to continuously learn from real-world applications post-distribution. In our era of rapid technological advancement, the incorporation of adaptive AI and its subset, machine learning (ML), has become pivotal in many SaMDs. This is attributed to the potential benefits AI/ML offers in deriving innovative insights from real-time data gathered during the product's everyday use in care settings.3
A significant development influencing the integration of AI/ML into medical devices is the emergence of LLMs. According to the U.S. FDA, LLMs are AI models trained on vast data sets, enabling them to recognize, summarize, translate, predict, and generate content tailored to specific prompts. The integration of LLMs promises to enhance diagnostic accuracy and optimize patient care delivery.
The impact of this innovation is far-reaching. Researchers at Stanford Medicine are at the forefront of AI medical research and study a wide range of potential uses of adaptive AI in medicine. As Curtis Langlotz, MD, Ph.D., and director of the Center for Artificial Intelligence in Medicine and Imaging, says, “AI can be, in some ways, superhuman because of its ability to link disparate data sources…It can take genomic information and imaging information and potentially find linkages that humans aren’t able to make.” This is just one of many applications adaptive AI can perform.4
However, researchers at Stanford are also aware of other pressing questions, including, how can AI be used responsibly in medicine? And subsequently, how will that impact the FDA requirements, whose primary oversight does not yet involve adaptive AI?
FDA Guidelines For Adaptive AI Medical Devices
Regulatory requirements and frameworks addressing the complexity of adaptive AI-driven medical devices are ongoing processes. As the field advances rapidly, stakeholders continually strive to establish comprehensive guidelines that accommodate the unique characteristics and challenges posed by this recent innovation. In the traditional manner, the FDA reviews medical devices and SaMD based on the appropriate pathways that the respective devices fall into. These pathways are not designed to fit adaptive AI/ML device technological models due to their progressive nature in making real-time improvements. The existing frameworks and pathways are tailored to monitor modifications in established devices, necessitating 510(k) submissions for changes. However, this poses a unique challenge for adaptive AI-driven devices, as they improve and make modifications in real time based on real-world usage. Determining the precise moment for such submissions becomes particularly complex.5
The FDA made significant strides in formulating strategic plans and drafting comprehensive guidelines for AI/ML-based medical products. In January 2021, the FDA published the AI/ML SaMD Action Plan, detailing the five actions in which the FDA intends to take to advance the agency’s oversight of AI/ML-based medical software. These actions include furthering their development in the proposed regulatory framework, supporting good machine learning practices and improving upon machine learning algorithms, promoting a patient-centered approach, developing methods to evaluate machine learning algorithms, and fostering efforts in real-world performance monitoring trials.6
Later the same year, the FDA published the Good Machine Learning Practice for Medical Device Development: Guiding Principles, which provided the 10 guiding principles regarding good machine learning practices and what to consider when developing AI/ML-based medical products. These principles are detailed further below:7
- Multidisciplinary Expertise Is Leveraged Throughout the Total Product Life Cycle: Understanding how the model fits into the clinical process, the advantages it offers, and any potential risks to patients can ensure the safety and efficacy of AI-driven medical devices.
- Good Software Engineering and Security Practices Are Implemented: The fundamentals of good software engineering practices, data quality assurance, data management, and robust cybersecurity practices are applied to ensure data authenticity and integrity as well as risk management.
- Clinical Study Participants and Data Sets Are Representative of the Intended Patient Population: Data collected should include characteristics (age, gender, and ethnicity) that are relevant to the intended population. This is to manage bias and allow the model to perform effectively while also highlighting any areas of limitation.
- Training Data Sets Are Independent of Test Sets: Training and test data sets are chosen to ensure they are independent, considering and addressing factors like patient details, data collection methods, and site variations to maintain this independence.
- Selected Reference Data Sets Are Based Upon Best Available Methods: Select the best methods to create a reference data set with well-defined and clinically relevant data to better understand any limitations of this reference. If available, using established reference data sets can ensure that the model works well across the target patient group.
- Model Design Is Tailored to the Available Data and Reflects the Intended Use of the Device: Create and follow robust test plans to evaluate device performance separately from the training data, including considerations regarding patient population, important subgroups, clinical environment, and use by the human-AI team, measurement inputs, and potential confounding factors.
- Focus Is Placed on the Performance of the Human-AI Team: The model involves human input in a “human in the loop,” approach, focusing on human factors and how easily the model’s outputs can be comprehended through a human-AI team, not just with the model in isolation.
- Testing Demonstrates Device Performance During Clinically Relevant Conditions: Develop and execute test plans to assess device performance separate from the training data, considering factors like patient groups, clinical settings, human-AI team interaction, measurement details, and possible influencing factors.
- Users Are Provided Clear, Essential Information: Users are given clear, contextually relevant information for the intended audience. This includes the product's intended use, performance for different groups, data details, limitations, and how the model fits into clinical workflows. Users are also informed about updates, decision-making basis, and ways to communicate feedback to the developer.
- Deployed Models Are Monitored for Performance and Retraining Risks are Managed: Deployed models are capable of real-world settings to ensure their safety and performance. When models are periodically or continually trained after deployment, safeguards are in place to prevent issues like overfitting, bias, or model decline that could affect their performance when used by the human-AI team.
Without government support and guidance, adaptive AI is set for a much more complicated course impacting patient health, safety, privacy, and beyond. Patient safety is addressed in President Biden’s Executive Order on the development and usage of AI in October of 2023:3
"[...] an HHS [Health and Human Services] AI Task Force that shall, within 365 days of its creation, develop a strategic plan that includes policies and frameworks — possibly including regulatory action, as appropriate — on responsible deployment and use of AI and AI-enabled technologies in the health and human services sector (including research and discovery, drug and device safety, healthcare delivery and financing, and public health), and identify appropriate guidance and resources to promote that deployment, including in the following areas: […] long-term safety and real-world performance monitoring of AI-enabled technologies in the health and human services sector, including clinically relevant or significant modifications and performance across population groups, with a means to communicate product updates to regulators, developers, and users;”
Also in October 2023, the FDA published the Predetermined Change Control Plans for Machine Learning-Enabled Medical Devices: Guiding Principles after garnering feedback from the public on the topic of the proposed regulatory framework for modifications to AI/ML-based SaMDs. This guidance document identifies five guiding principles for predetermined change control plans (PCCP). The term PCCP is defined by the FDA as “a plan, proposed by a manufacturer, that specifies certain planned modifications to a device, the protocol for implementing and controlling those modifications, and the assessment of impacts from modifications.” These guiding principles are:8
- The PCCP must be limited to modifications within the intended use or intended purpose of the original MLMD.
- The PCCP must be driven by a risk-based approach and align with the risk management approaches.
- PCCP is determined through evidence-based data, where the benefits outweigh any associated risks.
- PCCPs provide clear information that details plans for ongoing transparency to users and other stakeholders.
- PCCPs are used and developed from a total product life cycle (TPLC) perspective, meaning that the PCCP takes into account existing regulatory, quality, and risk management measures throughout the TPLC to ensure device safety.
On March 14, 2024, the FDA released Artificial Intelligence and Medical Products: How CBER, CDER, CDRH, and OCP are Working Together. This jointly published paper by the FDA’s Center for Biologics Evaluation and Research (CBER), Center for Drug Evaluation and Research (CDER), Center for Devices and Radiological Health (CDRH), and Office of Combination Products (OCP) represents their commitment to banding together to align and provide transparency regarding how FDA’s medical product centers are collaborating to safeguard public health while fostering responsible and ethical innovation. Managing adaptive AI medical technologies throughout their life cycle is a complex process, from initial ideas to maintaining functionality. It involves designing, getting the appropriate data, building and testing the model, deploying models, and keeping close monitoring to ensure ongoing performance, risk control, and regulatory compliance in real-world settings. A risk-based regulatory framework, supported by strong principles and tools, creates a flexible set of rules and tools to make sure this works across different medical products.9
References
- https://magazine.amstat.org/blog/2023/09/01/medicaldevices/#:~:text=Three%20examples%20
of%20AI%20CADt,of%20the%20brain%20or%20head - https://info.paige.ai/prostate
- https://www.fda.gov/medical-devices/software-medical-device-samd/artificial-intelligence-and-machine-learning-aiml-enabled-medical-devices
- https://stanmed.stanford.edu/translating-ai-concepts-into-innovations/
- https://www.fda.gov/files/medical%20devices/published/US-FDA-Artificial-Intelligence-and-Machine-Learning-Discussion-Paper.pdf
- https://www.fda.gov/news-events/press-announcements/fda-releases-artificial-intelligencemachine-learning-action-plan
- https://www.fda.gov/medical-devices/software-medical-device-samd/good-machine-learning-practice-medical-device-development-guiding-principles
- https://www.fda.gov/medical-devices/software-medical-device-samd/predetermined-change-control-plans-machine-learning-enabled-medical-devices-guiding-principles
- https://www.fda.gov/media/177030/download?attachment
About The Authors:
Jessica Chen is a recent Medical Product Development Management master’s of science graduate at San Jose State University. She holds a background in nursing, with a B.S. from the Valley Foundation School of Nursing at San Jose State University. Her interests lie within the medical device industry, particularly in the post-market surveillance sector, focusing on medical device vigilance, pharmacovigilance, and adverse event reporting. Chen has particular interest in improving patient safety and ensuring the effectiveness of medical devices through rigorous monitoring and reporting processes.
Thuha Tran has earned a master's degree in medical product development management at San Jose State University and holds a bachelor's degree in microbiology. She has experience in next-generation sequencing and project management for regulated medical devices. Highlights in her experience include supporting high-throughput sequencing pipelines, assisting in sensitive R&D experiments extracting and pooling DNA from live cells, and supporting the PMA approval for a cancer screening product. She currently works in project management for FDA- and EUMDR-regulated electrophysiology products.