AI-Driven Medtech Begins To Deliver On Its Potential
By Robert Hamilton, Ph.D., chief scientific officer and cofounder, NovaSignal
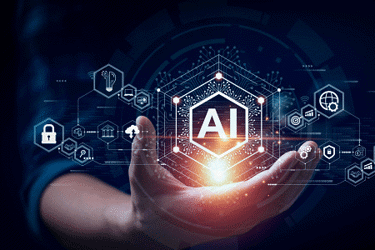
Every aspect of the healthcare industry has been impacted by technology, and further innovations will continue to advance the future of the industry. With the integration of artificial intelligence (AI) and machine learning (ML) into today’s medical devices, new insights gleaned from massive amounts of data have the potential to transform the use and delivery of healthcare. Today, healthcare professionals use AI-enabled devices and platforms every day, from performing robot-assisted joint replacements to analyzing X-rays and flagging anomalies for further review by a human radiologist. The use of AI is growing and expected to become a primary driver of medical device innovation globally.
A Long Time Coming
The successful use of AI in medicine reaches as far back as the 1970s. Researchers at Stanford University developed a program that identified bacteria that caused life-threatening infections such as meningitis. The program provided recommendations on the most effective antibiotic and dose for treating individual patients. The potential for AI and machine learning technologies to not only diagnose, but possibly even mitigate or prevent, serious disease has been clear ever since.
The next five decades brought advances in computing capacity, natural language processing (NLP), computer vision, and deep learning methodologies capable of processing high volumes of detailed image data. At the same time, the medical community was increasingly adopting health tech, like electronic health records, and achieving technological breakthroughs, like sequencing the human genome. The combination of these developments created fertile ground for the rise of AI-enabled medical devices.
Today, more than 530 AI/ML-enabled medical devices have been authorized1 by the FDA, with more added every quarter. The FDA recently released a new plan allowing developers of AI/ML-enabled medical devices to update those devices automatically and safely in response to new data. A clear sign of the growing acceptance of AI and ML, it will eliminate the current requirement to gain approval for every product update, which may be a lengthy process, and increases patient access to safe and effective advanced medical devices. With AI and ML, medical device researchers and manufacturers can use the latest available data to create new, and enhance existing, features designed to help doctors with their clinical decision-making process and improve patient care.
Promising Use Cases
The Mayo Clinic is applying AI to electrocardiogram (ECG) data captured by smartwatches. They developed an iPhone app that uses ECG data to identify patients with weak heart pumps. Another company designing AI-based solutions, Aidoc, can analyze X-ray images and flag positive cases of pneumothorax, or a collapsed lung. Lastly, the integration of AI-powered robots is revolutionizing the field of surgery.
In the fall of 2017, surgeons at Maastricht University Medical Center in the Netherlands used an AI-assisted surgical robot during microsurgery to suture small blood vessels in a patient affected by lymphedema. This robotic device leveraged AI to minimize any tremors in the surgeon’s movements, ensuring the device was correctly performing the procedure. Overall, the advances in imaging, analytics, sensor technology, and robotics are helping to drive AI forward to improve patient care.
The vast majority (75%) of AI-enabled devices and platforms are designed for radiology.2 Radiology is a science made for AI given the volume of highly structured data it generates. AI algorithms can improve image quality and perform initial triage to reduce potential errors or inconsistencies that can occur through human analysis of medical images.
For example, magnetic resonance imaging (MRI) provides highly detailed scans that enable physicians to see inside the body’s organs, bones, muscles, and nerves. However, these highly detailed images can be difficult for clinicians to interpret depending on their specialty or level of experience. Image quality can also vary from machine to machine.
GE Healthcare developed a solution that mitigates these challenges through a deep-learning reconstruction algorithm that quickly provides radiologists with crisp MRI images by improving the signal-to-noise ratio in the raw image data. In essence, the algorithm prevents flaws such as ghost images caused by patient movement, signal processing errors, or hardware-related faults. This not only results in higher-quality images, but also reduces scan times by up to 50%, which improves the radiologists’ workflow, increases the number of patients a facility can accommodate, and most importantly, enhances the patient experience.3
For decades doctors have used transcranial Doppler ultrasound (TCD) to evaluate and measure the rate and direction of cerebral blood flow. While it is non-invasive, painless, and an important device to monitor brain circulation, TCD requires trained technicians to find a very thin spot in the skull that the ultrasound can penetrate. According to industry data, fewer than 5%4 of U.S. ultrasound technicians are trained in manual TCD, meaning very few hospitals offer this often lifesaving diagnostic. By integrating AI-guided robotics and automation into the device, it suddenly becomes a tool that healthcare professionals can use even if they have not had specialized TCD-training.
As an example, vascular neurologist Mark N. Rubin, MD, of Edward Hines Jr. Veterans Affairs Hospital in Illinois, is presenting data at the 2023 American Academy of Neurology annual meeting on leveraging an AI-driven robotic-assisted transcranial Doppler (raTCD) versus transthoracic echocardiography (TTE) for right to left shunt (RLS) detection. A right to left shunt is a recognized risk factor for stroke. The multi-site retrospective review of real-world clinical data using raTCD for RLS diagnosis did not differ from the data presented previously showing the robotic-assisted TCD device was three times more likely to diagnose RLS than current standard of care TTE. This supports the notion that the AI-driven robotic-assisted device may allow healthcare providers to achieve the known sensitivity of TCD for RLS detection without the need for an experienced operator to perform the examination in routine clinical practice.
Conclusion
Forecasters estimate that total global spending on healthcare AI will reach more than $185 billion in 20305 with the medical and technology industries approaching the opportunity from all angles. From chipmakers like NVIDIA to industry leaders such as Google Health and scrappy startups working in stealth, the industry has just scratched the surface of how AI, ML, and robotics can assist the medical device industry.
References
- Artificial Intelligence and Machine Learning (AI/ML)-Enabled Medical Devices | FDA
- The Advent of Artificial Intelligence & Machine Learning in Medical Devices (qbdgroup.com)
- https://www.gehealthcare.com/products/magnetic-resonance-imaging/air-technology/air-recon-dl
- Internal analysis based on industry data
- AI in healthcare market size worldwide 2030 | Statista
About The Author:
Robert Hamilton, Ph.D., is chief scientific officer and cofounder of NovaSignal. He is an entrepreneur, engineer, and clinical researcher with a passion for innovative technologies in brain health management. He is an expert in image/signal processing and machine learning, with extensive experience in cerebral blood flow, traumatic brain injury, stroke, and other neurological disorders.