AI In Medical Devices: Meeting The Regulatory Challenge Around The World, Part 2
By Marcelo Trevino, independent expert
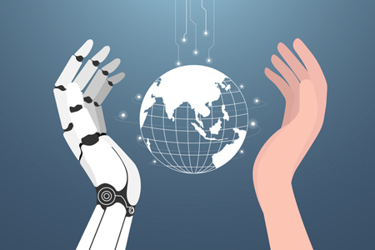
In Part 1 of this series, I explored how countries across the globe are shaping regulatory frameworks to manage the rapid integration of artificial intelligence (AI) and machine learning (ML) in medical devices. This has resulted in a complex, dynamic regulatory landscape that’s evolving in real time.
Now in Part 2, I will focus on the common regulatory threads that connect different regions and the global standards and principles being developed to bring consistency, safety, and trust to AI innovation in healthcare. From good machine learning practice (GMLP) principles to ISO and NIST frameworks, the world is moving toward a shared language around AI, one that balances innovation with patient protection.
I will also cover the practical, forward-looking steps that companies should be taking now to thrive in this new environment. Whether you're preparing submissions for global markets or embedding AI into your product road map, this guide will help you anticipate requirements, reduce risk, and lead with confidence.
International Medical Device Regulators Forum (IMDRF): Driving Global Convergence Through GMLP
As AI becomes more embedded in medical devices, the need for international alignment on regulatory expectations has never been more urgent. Enter the International Medical Device Regulators Forum (IMDRF), a voluntary group of medical device regulators from around the world, working together to harmonize approaches to emerging technologies, including Software as a Medical Device (SaMD) and AI/ML-enabled systems.
The IMDRF plays a pivotal role in shaping a common regulatory vocabulary and set of principles for AI in healthcare. One of its most impactful contributions to date is the promotion and co-development of GMLP, a framework designed to ensure that AI systems in medical devices are not only innovative but also safe, effective, ethical, and trustworthy throughout their life cycle.
The GMLP principles, jointly developed by the U.S. FDA, Health Canada, and the U.K.’s MHRA, serve as a blueprint for manufacturers and regulators alike. They’re not just technical checklists, they reflect a holistic view of how AI should be developed, validated, and deployed responsibly.
Key Pillars Of GMLP And Their Global Significance
1. Multidisciplinary Expertise from the Start: GMLP emphasizes the need for diverse, cross-functional teams to be involved from the earliest stages of AI system development. This includes not just software engineers and data scientists but also clinical experts, quality assurance leads, and regulatory professionals. The rationale is simple: by bringing in multiple perspectives, companies can identify risks earlier, design more usable systems, and ensure the technology supports real-world clinical workflows.
2. Data Quality, Diversity, and Representativeness: One of the biggest risks in AI systems is bias. GMLP requires developers to carefully consider the origin, structure, and balance of their training data sets. This means using data that is representative of the intended patient population across demographics, geography, and clinical variability. Developers must document their data sources, justify inclusion/exclusion criteria, and apply techniques like stratification or augmentation to address underrepresented groups. High-quality, representative data is not just a best practice, it’s foundational to fairness and clinical validity.
3. Model Transparency and Explainability: AI doesn’t belong in healthcare if clinicians can’t trust it. GMLP insists on interpretability, so AI systems must be designed in ways that make their outputs understandable to end users and acceptable to regulators. This includes not only confidence scores and traceable decision pathways but also thorough documentation of model architecture, training workflows, and performance metrics. In other words, the “why” behind an AI recommendation should never be a mystery.
4. Continuous Performance Monitoring and Post-market Evaluation: Unlike traditional devices, AI systems are dynamic, and they can evolve, degrade, or drift over time. GMLP requires a robust post-market monitoring plan that includes regular audits, real-time performance tracking, and mechanisms to detect when the algorithm’s behavior starts to deviate from its validated baseline. This ensures that safety and effectiveness aren’t just proven once but maintained continuously. Feedback loops, from users, real-world data, and adverse events, must be built into the quality system to inform timely updates and retraining.
5. Ethical and Human-Centered Design: Perhaps one of the most distinctive aspects of GMLP is its focus on ethics and human-in-the-loop systems. AI in healthcare should enhance, not replace clinical decision-making. That means systems must include clear override options, communicate uncertainty, and support clinicians in high-stakes environments. GMLP underscores that patient trust and clinical judgment are nonnegotiable, and that technology should be built around the needs and limitations of real users, not just code.
The Strategic Value Of Aligning With GMLP And IMDRF
For companies seeking to market AI-enabled devices across multiple regions, aligning with GMLP and IMDRF recommendations isn’t just wise, it’s strategic. These principles are becoming the unofficial foundation upon which many national regulatory frameworks are being built. Countries like the U.S., Canada, and the U.K. are directly embedding GMLP into their guidance documents, and other regions are beginning to reference these practices as part of their evolving regulatory expectations.
Adopting GMLP early in development can help streamline submissions, reduce review times, and demonstrate a proactive commitment to global safety and quality standards. It signals to regulators that a manufacturer understands not only the technical requirements of AI but also the ethical and societal responsibilities that come with deploying it in clinical care.
In short, GMLP is more than a checklist; it’s a compass for responsible AI innovation in healthcare.
Standards Bodies: Laying The Foundation For Trustworthy AI In Healthcare
As regulators work to catch up with the rapid advancement of AI and ML technologies, international standards organizations are playing a crucial role in providing a structured, harmonized foundation for safety, quality, and risk management. These standards serve as the technical scaffolding that supports regulatory frameworks and industry best practices around the world.
By aligning with established standards from organizations like ISO, IEC, AAMI, and NIST, companies can proactively demonstrate compliance, reduce regulatory friction, and build trust with authorities, clinicians, and patients. Let’s take a closer look at the most influential standards shaping AI in healthcare, and why they matter.
- ISO/IEC 23053 – Framework for AI Systems Using ML: This international standard provides a comprehensive life cycle model for the development and maintenance of machine learning-based AI systems. It maps the phases of AI system evolution, from initial design and training to deployment, monitoring, and retirement. AI is not a “set-it-and-forget-it” technology. ISO/IEC 23053 encourages structured thinking about how AI systems evolve over time and how to embed controls at every stage of the process. It supports sustainable innovation by ensuring that changes, retraining, and post-market adjustments are deliberate, validated, and documented.
- ISO/IEC 42001 – Artificial Intelligence Management System (AIMS): This is the first AI-specific quality management system (QMS) standard, released to help organizations establish the governance, accountability, and process discipline necessary for trustworthy AI. Key elements include:
- AI governance structure
- risk and impact assessments
- transparency and responsibility frameworks
- documentation and continuous improvement mechanisms.
Medical device manufacturers are already familiar with ISO 13485 for QMS. ISO/IEC 42001 builds on that familiarity but is tailored for the unique needs of AI/ML development, including ethical considerations, model explainability, and stakeholder engagement. For companies integrating AI into their medical products, AIMS becomes a critical overlay that ensures systems are governed responsibly from a management and organizational perspective.
- ISO/IEC 23894 – Artificial Intelligence – Risk Management: This standard offers guidance for identifying, assessing, and managing the unique risks associated with AI, such as algorithmic bias, unintended outputs, and misuse. It emphasizes:
- risk identification across all AI life cycle phases
- mitigation strategies tailored for data-driven systems
- transparent reporting and documentation of residual risks
- integration of risk management with organizational controls.
Risk in AI goes beyond physical harm; it includes systemic bias, erosion of trust, and opacity in clinical decision-making. ISO/IEC 23894 helps companies extend traditional device risk management frameworks to encompass these digital and ethical dimensions.
- AAMI CR34971 – Guidance on ISO 14971 Application for AI/ML in SaMD: Published by the Association for the Advancement of Medical Instrumentation (AAMI), this consensus report adapts the well-known ISO 14971 risk management process specifically for AI/ML-enabled Software as a Medical Device (SaMD). It provides:
- practical interpretations of ISO 14971 in the context of AI
- recommendations for managing adaptive behavior and retraining
- risk controls for data quality, algorithm drift, and user misuse
- examples of harm scenarios unique to AI in healthcare.
ISO 14971 is a cornerstone of medical device safety. CR34971 bridges the gap between that legacy standard and the new realities of software-driven, adaptive devices, offering a pathway for companies to apply familiar risk practices to unfamiliar AI systems.
- NIST AI RMF 1.0 – Artificial Intelligence Risk Management Framework: Developed by the U.S. National Institute of Standards and Technology (NIST), this framework focuses on making AI trustworthy, secure, and ethically sound. While not limited to healthcare, its principles are directly applicable to AI used in medical technologies. The framework is organized around four core functions:
- Govern – Establish structures to manage AI risk.
- Map – Understand contexts and intended uses.
- Measure – Assess and monitor performance, bias, and security.
- Manage – Prioritize risks and implement control strategies.
The NIST AI RMF provides a nonprescriptive, flexible toolkit that organizations can use to tailor their risk posture based on context, criticality, and exposure. It is particularly useful for companies operating in or entering the U.S. market and for those seeking to build an internal culture of trustworthy AI development.
The Strategic Value Of Aligning With Standards
In today’s fragmented regulatory environment, adherence to recognized standards isn’t just a matter of good engineering practice, it’s a competitive advantage. Standards:
- Reduce regulatory uncertainty by aligning with what authorities expect
- Accelerate approvals by providing pre-validated frameworks for safety and risk
- Promote interoperability, consistency, and audit readiness
- Help organizations scale AI initiatives across markets with minimal rework
- Enhance transparency and trust among clinicians, patients, and partners
For companies developing AI-enabled medical technologies, aligning with ISO, IEC, AAMI, and NIST standards demonstrates not only regulatory maturity but a proactive commitment to responsible, human-centered innovation. In a world where AI is redefining what medical devices can do, standards help ensure we don’t lose sight of what they should do: improve lives safely, equitably, and ethically.
Cross-Cutting Themes In Global AI/ML Regulation And How Medical Device Companies Can Prepare Now
As AI and machine learning become integral to modern healthcare, a set of universal regulatory expectations is beginning to take shape. While countries differ in their specific requirements and documentation formats, there is growing global convergence around key principles that define what trustworthy, safe, and effective AI in medical devices should look like.
These cross-cutting themes are forming the backbone of global AI/ML oversight, and companies that embed them early will be in the strongest position to scale, adapt, and lead in this space.
1. Life Cycle Oversight, Not Static Approvals
Traditional medical device approvals were designed for fixed-function technologies; they were products that remained unchanged once cleared for market. But AI doesn’t work that way. Algorithms evolve, adapt, and in some cases, retrain themselves in response to real-world data.
Global regulators now expect continuous oversight of AI performance across the entire product life cycle. This includes real-time monitoring for model drift, retraining thresholds, risk flagging systems, and audit trails to ensure changes remain safe and clinically valid.
How medical device companies can prepare now: Embed AI-specific oversight processes into your QMS. Define performance metrics, set acceptable ranges, and build escalation protocols that trigger retraining, rollback, or risk evaluation. This ensures regulatory readiness and patient safety, long after market entry.
2. Human Oversight and System Explainability
Across every jurisdiction, regulators are aligned on one nonnegotiable principle: AI should never operate as an unchecked black box. If clinicians can’t understand or trust an AI system’s recommendation, they’re unlikely to use it, and regulators are unlikely to approve it.
Explainability is now a baseline expectation. Systems should communicate how decisions are made, how confident the model is, and what its limitations are. Human override must be built in, allowing users to interpret or challenge outputs when necessary.
How medical device companies can prepare now: Incorporate explainability during the design phase, not as an afterthought. Develop intuitive interfaces that display decision rationales, uncertainty scores, and visual indicators. Educate end users on how to engage with the system and when to override or escalate concerns.
3. Strong Data Governance and Cybersecurity by Design
Data is the fuel that powers AI, and poor data governance can compromise everything from accuracy to equity. Regulators now expect companies to demonstrate that training data sets are ethically sourced, representative of the intended population, and securely managed.
In parallel, AI systems are increasingly connected to hospital networks, cloud platforms, and real-world devices, raising serious cybersecurity concerns. The risk of breaches, manipulation, or service disruption must be anticipated and mitigated at every stage.
How medical device companies can prepare now: Establish a robust data governance framework: document sources, inclusion/exclusion criteria, cleaning methods, and representativeness. Implement encryption, access controls, breach response protocols, and regular vulnerability assessments. Stay aligned with global privacy laws like GDPR, HIPAA, and emerging AI-specific security standards.
4. Global Convergence with Local Nuances
While the push toward international harmonization is accelerating, driven by IMDRF, GMLP, ISO standards, and shared risk frameworks, each country or region still maintains its own nuances. Documentation, terminology, submission format, and expectations can vary significantly. Companies pursuing global commercialization must embrace a “core + localization” model: build a global regulatory foundation and adapt it to regional requirements without starting from scratch each time.
How medical device companies can prepare now: Develop modular regulatory dossiers that include shared content (e.g., risk management, data strategy, explainability) and allow for local customization. Assign a global regulatory intelligence team to track evolving rules and ensure continuous alignment.
Practical Steps For AI-Enabled Medtech Companies To Prepare Now
To stay ahead of regulatory scrutiny while accelerating innovation, medical device organizations can take the following proactive steps:
- Appoint a Cross-Functional AI Compliance Team: Establish a team that spans regulatory affairs, clinical, data science, legal, software, and quality. This ensures that AI strategy is integrated across design, risk, and market access planning, from early concept to post-market surveillance.
- Conduct a Portfolio-Wide Risk Assessment of AI-Enabled Products: Identify which products or features contain AI or ML elements. Classify their risk levels based on intended use, clinical impact, and degree of automation. Use this insight to prioritize documentation, redesigns, or regulatory engagement.
- Build Comprehensive AI Technical Documentation: Create detailed, audit-ready documentation covering your training data, model development workflows, algorithm logic, validation methods, and update mechanisms. Include transparency tools such as model rationales, traceability matrices, and explainability statements.
- Establish Structured Change Management and Retraining Protocols: Define what types of changes are expected post-market (e.g., algorithm refinements, expanded indications) and when retraining should occur. Document how updates are tested, validated, and approved, internally and for regulatory resubmission.
- Educate Users on System Capabilities and Override Controls: Train clinical users on how to interpret outputs, what the system can and cannot do, and how to intervene when necessary. Build formal feedback mechanisms to capture user input and inform post-market improvements.
- Monitor Global Guidance and Adapt Your QMS: Designate a function or platform to stay on top of evolving regulatory guidance in all key markets. Incorporate AI-specific considerations into existing QMS processes such as design controls, CAPA, complaint handling, risk management, and post-market surveillance.
In this dynamic regulatory landscape, preparedness is not a luxury – it’s a strategic necessity. The companies that thrive in the AI era will be those that embrace these cross-cutting principles early, build with transparency, and operationalize compliance as a driver of both trust and innovation.
Conclusion
As we move deeper into the AI era, it's clear that innovation alone isn’t enough; responsible innovation is the new gold standard. Around the world, regulators and standards bodies are laying the groundwork for safe, effective, and ethical AI systems that can transform healthcare without compromising trust or safety.
For companies developing or deploying AI-powered medical devices, this means compliance must become part of the innovation process, not a checkpoint at the end. It also means staying agile: monitoring global guidance, aligning with evolving standards like ISO 42001 and GMLPs, and building systems that are explainable, secure, and inclusive by design.
Success in this next chapter won’t come from speed alone. It will come from those who build AI with foresight, cross-functional collaboration, and a deep commitment to patient impact. Regulatory professionals, now more than ever, have the opportunity to lead, not just in managing risk but in shaping the future of ethical, scalable AI in healthcare. The time to act is now, as the road map is forming. And those who prepare today will shape tomorrow.
About The Author:
Marcelo Trevino has more than 25 years of experience in global regulatory affairs, quality, and compliance, serving in senior leadership roles while managing a variety of medical devices: surgical heart valves, patient monitoring devices, insulin pump therapies, surgical instruments, orthopedics, medical imaging/surgical navigation, in vitro diagnostic devices, and medical device sterilization and disinfection products. He has an extensive knowledge of medical device management systems and medical device regulations worldwide (ISO 13485:2016, ISO 14971:2019, EU MDR/IVDR, MDSAP). He holds a BS in industrial and systems engineering and an MBA in supply chain management from the W.P. Carey School of Business at Arizona State University. Trevino is also a certified Medical Device Master Auditor and Master Auditor in Quality Management Systems by Exemplar Global. He has experience working on Lean Six Sigma Projects and many quality/regulatory affairs initiatives in the U.S. and around the world, including third-party auditing through Notified Bodies, supplier audits, risk management, process validation, and remediation. He can be reached at marcelotrevino@outlook.com or on LinkedIn.