Automation vs. Artificial Intelligence In Medtech: Where Are We, And Where Are We Going?
By Atif Nawaz, GlobalData
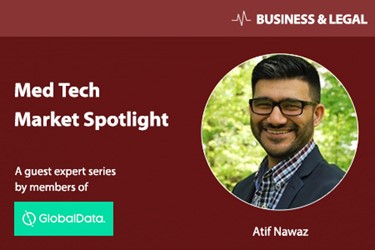
There is a significant difference between automation and the use of artificial intelligence (AI) in the medtech space. Automation, within a healthcare setting, is defined as the use of hardware and software specifically programmed to save time. AI can be categorized as machine learning, meaning software and hardware working in conjunction to effectively mimic human decision-making — just much, much faster. AI can learn outside of its programming, and the goal is for the software to make a decision of equivalent quality, compared to a human.
The use of automation and AI is integral within the medtech space, as data is becoming increasingly important to manage and understand. Data management is a multifaceted issue within the healthcare industry, but understanding and effectively utilizing it can lead to better patient outcomes. In addition, automation can save man-hours by replacing menial — and some intricate — tasks, allowing health practitioners to focus on more important tasks.
The Case For Automation
Currently, automation’s principal medical device-related use serves this very objective: to free up healthcare practitioners’ time to focus on more complex tasks that require a higher level of thinking. As data management becomes a prime directive for hospitals, clinics, and labs, there are numerous solutions to create a holistic solution for patients.
A paragon of automation is Electronic Health Records (EHR) within the healthcare space; not only do these programs have full patient profiles readily available, they can also incorporate patient tests — such as MRIs, X-Rays and in vitro diagnostics — directly onto the record from testing departments. This allows patients to move departments within a hospital while their full record follows them for easier clinical decision-making. However, EHR management programs in medtech suffer from low connectivity to other EHR management systems, as well as a lack of interoperability.
Fig. 1 — Forecasted revenue breakdown for Healthcare IT within healthcare settings in 2018.
Fig. 1 illustrates the importance of clinical information technology systems within a healthcare setting. These clinical IT systems represent effective use of large-scale automation within the healthcare industry. Fig. 2 further breaks down clinical IT systems, of which more than 60 percent of revenue is generated by EHR systems.
Fig. 2 — Forecasted revenue breakdown of Clinical IT systems within a healthcare setting in 2018.
EHRs involve large data sets, which automation makes easier to manage. Thus, the focus within the last few years has been on streamlining large amounts of patient data, with EHR systems becoming the most common type of automation in healthcare facilities. Conversely, a smaller segment of automation is applied to robotic surgery.
Currently, robotic surgery is a type of automation, rather than the use of AI, as it merely assists human surgeons during specific surgical procedures. Robot-assisted surgery grants surgeons greater mobility while within minimally invasive procedures. “Using CT and MR images, as well as GPS for target localization, is more precise than the human hand-eye coordination of the surgeon alone,”1 states Garnette Sutherland, professor of neurosurgery at the University of Calgary.
Automation, meanwhile, has become a health industry mainstay due to healthcare facilities trying to maximize throughput and increase efficiency while reducing overall expenditures. Automation not only saves time and some costs associated with manual labor, but removes hindrances that affect patient outcomes. Another example of automation applied to healthcare is wound-measurement companies, where automated tasks supersede conventional wound-measuring techniques.
Traditionally, a wound is measured by hand; health care workers use rulers to record the width, length, and depth of the wound. However, lack of consistency in measurements may alter a clear indication of progress in the wound healing process. Automating this task removes human diagnostic bias, thus making quantitative results more accurate. Similarly, AI is currently used in diagnostic measures effectively, as algorithms can be used to detect anomalies that may be missed by humans; plus, the work is completed within a fraction of the time.
Automation is heavily evident and relied upon currently within the healthcare industry. However AI has significantly higher potential within the health space in shifting the paradigm to streamline work flows and create better patient outcomes. AI is effectively used in diagnosis, personalized medicine, treatment program development, drug development and patient monitoring. AI consistently learns to mimic human cognitive functions in order to visualize trends within large sets of medical data.
AI In Healthcare Systems
A recent partnership between University College London Hospitals (UCLH) and the Alan Turing Institute sparked the adoption of AI within the National Health Service (NHS). The end goal of the partnership is to increase AI adoption to detect and triage patients with critical emergency ailments. AI machine learning algorithms will be used to detect patients registered with benign indications, such as abdomen pain, and to detect severe issues, such as systemic infections or intestinal proliferation2. There are various programs being implemented by the Alan Turing Institute in UCLH institutions.
The Canadian government, too, is cognizant of the role automation and AI will play within its burdened healthcare system. The Medical Device Regulations under Health Canada have approved and issued attainable licenses for AI innovations within the country,1 with 14 regulatory recommendations made to incorporate AI into the Canadian healthcare system.
As more nations become aware of AI integration within healthcare settings, the correct regulatory frameworks, in conjunction with large international companies, will revolutionize healthcare globally.
Freenome, Raysearch, and IBM Watson Health, as well as Microsoft Project Hanover and Google Deepmind, are just a few of the companies with AI initiatives in the healthcare space. However, the distinction between automation and AI becomes apparent in the technology each company is focused on. Fig. 3 illustrates the relationship between automation and AI; low automation and low AI are commoditized medical devices. The market is dominated by automation companies, as hospitals and health institutions need to keep costs at a minimum while increasing data management and automating repetitive tasks.
Fig. 3 — Qualitative measure of some companies increasing the level of automation and AI in healthcare
The main focus of automation and AI startups is to gain traction with established manufacturers within the medtech space. As implementation within health settings for new technologies can be hindered by slow adoption, partnering with large multinationals can circumvent these hindrances. For example, two of the smaller AI companies listed above — Freenome and Raysearch — both entered into deals with larger entities (a diagnostics firm and a medical center, respectively) to speed development and adoption of their products. Transcriptic and Five Prime Therapeutics, meanwhile, are among the automation firms partnering with larger entities toward that end.
Larger companies — such as Roche, Qiagen, and Eli Lilly — can aid in providing the infrastructure needed for automation and AI to be adopted within healthcare settings, as well as provide a foot in the door with healthcare systems. Such strategic partnerships not only create better patient outcomes, but increase revenues for all stakeholders as newer technology sees higher adoption rates.
Regulation
As more automation and AI systems are incorporated into the healthcare space, regulation will be a limiting factor for progress. Tech companies currently are not hampered by regulation when trying to innovate, but, once patients become involved, regulatory bodies cannot take chances that could lead to adverse events, most notably loss of life.
In 2017, the FDA implemented the Digital Health Innovation Plan (DHIP) to safely integrate digital health products into the market4. The DHIP is an initial step towards the regulation of automation devices, which will eventually translate to AI regulation by the FDA. Within the DHIP, the FDA launched a Software Precertification Pilot program, which enrolled major tech giants in order to categorize cloud-based healthcare solutions into a conventional regulatory medical device framework.
Digital programs dealing with patient information also must comply with privacy stipulations in the Health Insurance Portability and Accountably Act (HIPAA), as well as similar regulations mandated by foreign regulatory bodies. For example, the HIPAA equivalent in Canada is the Personal Protection and Electronic Documents Act (PIPEDA).
Future Outlook
Automation is widespread within healthcare, with AI to follow as more companies move towards AI development and integration. Eventually, this will lead to AI systems being layered on top of automated devices, leading to intelligent automation.
Intelligent automation will combine the prowess of automation with the cognitive abilities of AI. As more stakeholders are involved with automation, AI, and general data management within a healthcare context, the move towards a combination of all three aspects will create healthcare solutions equivalent to sci-fi aspirations.
About The Author
Atif Nawaz is a Medical Device Analyst for GlobalData Healthcare across multiple therapeutic areas. Within GlobalData, Atif has experience with the Wound Care, General Surgery, and In Vitro Diagnostics markets, working on market assessment and forecast, including market models and reports. His academic experience includes quantitative and qualitative research methods, health information management, and public health. Prior to joining GlobalData, Atif worked as an Analyst at ConMed Canada and developed an expertise in orthopedics and general surgery while working on ad-hoc projects and liaising with the sales team. Atif holds a BSc (Hons) from the University of Ontario.References
- Standing Senate Committee on Social Affairs, Science and Technology (Oct, 2017). CHALLENGE AHEAD Integrating Robotics, Artificial Intelligence and 3D Printing Technologies into Canada’s Healthcare Systems.
- UCLH. Revolutionising healthcare with AI and data science.
- GlobalData Medical Intelligence Center. Companies Database (available via subscription)
- US Food & Drug Administration (2017). Digital Health Innovation Action Plan.