Challenges Implementing New Advanced HFE testing Methods
By Charles L. Mauro, Mauro Usability Science
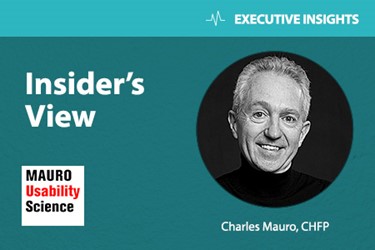
This is the second in a two-part series taking a hard look at current HFE testing methods and examining new advanced testing methods that HFE teams can utilize to improve the HFE performance of medical devices. Read part one here.
Part one of this series presented neuroscience-based HFE data capture methods that are beginning to penetrate HFE testing programs, and are being combined with traditional observational research to produce unprecedented levels of HFE device performance data. This installment will discuss the challenges of utilizing advanced data capture systems in real world HFE testing programs.
It is no surprise that the creation, testing, calibration, verification, and maintenance of an advanced HFE testing framework — that is cost-effective and usable in real-world HFE testing programs — is both complex and challenging. When undertaking such a development effort, one encounters several core problems that require significant intellectual and financial capital. The benefits of committing resources to this endeavor also are discussed, and some are surprising.
Several factors impact successful transition to these new methods. The following discussion of special challenges is based on creation and utilization of a fully operational advanced HFE system, which successfully combines many of the advanced methods discussed in part one, including: 3D spatial tracking, EEG, EMG, high-performance eye-tracking, Newtonian force measurement, micro-facial expression analysis, and GSR combined with advanced statistical analysis.
The system described has been utilized in over 200 real-world HFE testing programs focusing on medical device and IFU optimization efforts, and it has been applied to medical devices ranging from simple to highly complex, multi-modal user interfaces — combining hardware, software, and complex training procedure development. These are the lessons learned.
Staggering Amounts Of Data Are Produced And Must Be Integrated
A typical advanced HFE study, combining several of the methods discussed above, produces several gigabytes of raw data that must be subjected to analysis and summarization. The sheer amount of data demands a robust data capture framework designed to specifically address this challenge. New data capture methods produce the HFE study equivalent of “big data” and, as such, demand an entirely new way of thinking about study design, data acquisition design, analysis, and reporting.
The single most complex problem when utilizing these new HFE testing methods is integration of all the various data sets into a unified data stream that can be utilized to understand what actually is taking place during a given product’s HFE analysis. This can be a particularly difficult problem, given that each sensing modality produces different output file formats, sampling rates, and time stamping functions.
Addressing the integration problem requires development of specialized software and data integration systems designed and implemented with medtech in mind. Without a robust and verified data integration system, it is nearly impossible for HFE research teams to conduct advanced studies in a manner that is cost effective and timely.
Any new data integration systems must be carefully pilot tested across all modalities to ensure proper functioning, and to maintain the integrity of the combined data stream. Simply purchasing a set of advanced testing hardware and plugging it into a data integration platform will not produce a system that begins to deliver reliable and repeatable data capture. For context, consider that the system discussed in this article required roughly six years of research and verification testing.
System Calibration And Standard Operating Procedure Must Be Developed
As data capture systems provide finer levels of HFE performance metrics, it becomes essential that all systems be professionally maintained and calibrated. This is a non-trivial problem, as many of the data capture methods described above must be constantly updated, maintained, and calibrated before, during, and after all critical HFE testing sessions. This is a fundamental requirement, and one that drives the need to develop rigorous standard operating procedures (SOPs) and related internal system management processes designed to ensure data capture and data integration is accurate and reliable.
Because of this constant maintenance, an HFE research team cannot rely on data capture system vendors to ensure calibration and overall system performance. For HFE research teams engaged in submission of data to the FDA, calibration and SOP development must be developed in-house, where the FDA’s demands are better understood. This complicates the team structure and adds overhead to HFE testing programs that must be factored into study design, as well as research budgeting and timing.
HFE Research Expertise Must be Secured
These new methods require HFE expertise that normally is not part of an HFE research staff’s education and professional experience, be it design, execution, or reporting of the data capture methods described above. This leads to a need for specialized training in system utilization, but, more importantly, training in data interpretation.
More advanced combinations of these HFE testing methods commonly require HFE testing teams composed of experts with very specific educational and academic experience, with a clear focus on cognitive neuroscience. However, this challenge also adds a major benefit, as the composition of HFE research teams expands to provide far more rigorous and science-based insights, based on team composition.
Statistical Analysis Gains Increased Importance
With such a dramatic increase in the amount of data, and the need to integrate such data into a common data stream, comes the opportunity to utilize advanced statistical methods. This means that HFE research — normally highly subjective and based on small subject samples — can provide reliable insights supported by large data sets, providing objective measurement of HFE performance within and between medical device design options, patient populations, and IFU configurations. The inherent challenge is that HFE research staff must understand which statistical methods can and should be applied to make use of new data on HFE performance.
In some applications where advanced methods are utilized, it is necessary to draw upon the expertise of individuals with advanced training in study design and statistical analysis. There also is a requirement that the use of advanced statistical methods be executed on a reliable and approved stats package that may or may not be CFR21 Part 11 compliant. All statistical packages utilized in data analysis must, by definition, accept export frameworks flowing from the data integration platform.
These new data capture methods produce streaming data in a wide range of file types and data rates. This can and does dramatically complicate data integration and data analysis. It turns out that this problem extends well beyond simple time-synching data streams and extends to data export and import functions not commonly found in traditional HFE testing data sets, which tend to be very simple and, for the most part, text-based.
Custom Hardware / Software Must Be Developed And Supported By A Stable Vendor
Development and deployment of advanced HFE testing methods requires development of custom software and hardware. This is common practice when these solutions are utilized in clinical applications, covering both industry and academic settings. The primary challenge with the creation of such solutions is the formal requirement that such software and hardware solutions comply with various FDA and industry standards in terms of quality assurance and functional verification.
Further complicating this challenge is the fact that most of the new advanced data capture methods have been developed by smaller companies, which specialize in a given sensing technology or clinical application. These same companies can be relatively weak in terms of customer support, and on occasion fail to update their system in line with operating system updates. This is a challenge that requires constant monitoring on the part of the HFE research team utilizing these new data capture methods.
In some instances, data capture system manufacturers cease operation or are acquired by larger entities, which may drop entirely the very sensing system a medtech uses as part of its larger data capture system. HFE research professionals recently experienced this problem when Apple acquired SMI — a developer of advanced eye-tracking technology — and stopped producing and supporting SMI eye-tracking systems. This critical concern impacts system reliability and replacement costs.
Methodology Verification And Pilot Studies Must Evolve
Advanced HFE testing methodologies cannot be simply adopted and integrated into patient/device testing programs without careful and professional verification of both the stability and quality of the sensing technology. Such verification requires sometimes extensive bench and pilot testing to determine if the new methodology is actually delivering data in a manner that produces reliable metrics. The problem is made more complex by the continuous introduction of new sensing and data capture methods into the global research marketplace. This dramatically complicates the selection and adoption of a new HFE data capture methodology that will “play nice” with the other sensing systems, which, by necessity require integration into the unified data stream. It is also well known that companies that develop advanced sensing and data capture systems sometimes overpromise and under-deliver.
There are significant challenges associated with the creation and implementation of an advanced HFE testing framework that requires significant financial and intellectual resources. However, the benefits when such systems are in place are remarkable and potentially industry changing.
Conclusions
Based on the use of combinations of these advanced testing methods in over 200 projects, covering a wide range of drug delivery devices, IFU development programs and medical product development initiatives, it is clear that modern advanced testing methods have significant benefits for those striving to create improved clinical outcomes based on improved HFE performance of medical devices and related technology-based systems.
However, these new methods do not replace traditional observational research; they act as a source of verification and expansion, leading to a deeper understanding of actual HFE performance for a medical device or system. Traditional observational HFE research must always work in tandem with these new systems to ensure the best possible outcomes for all involved, including those who create, approve, and utilize increasingly powerful medical devices and related patient interaction frameworks.
About The Author
Charles L. Mauro, CHFP is Founder and President of MAURO Usability Science, a New York-based consulting firm founded in 1975 specializing in advanced human factors engineering research and the optimization for complex medical devices and related technology. He is Chairman of the Design Protection Section for the Industrial Designers Society of America and is the IDSA liaison to the US Patent and Trademark Office. He has lectured on product design and human factors engineering research at MIT, Stanford University, UPenn, and many other leading human factors research and engineering programs. Over his 47-year career Mr. Mauro has managed over 4,000 HFE research and development projects.
Mr. Mauro holds a BS with distinction in Industrial Design from The Los Angeles Art Center College of Design and a Master’s Degree in Ergonomics and Biomechanics from New York University. At NYU he was an appointed NIOSH Research Fellow at the RUSK Institute of Rehabilitation Medicine. His experience with complex medical technology spans more 47 years.
Reviewers: The author would like to thank the following reviewers. The content of this article is based solely on the opinions of MAURO Usability Science and, specifically, the author. It may not reflect opinions of the reviewers or their organizations.
- Conne Mara Bazley, Ph.D., CHFP, Principal Human Factors Engineer Pfizer, Inc.
- Brendan Murray, VP Enablement Services, iMotions, Inc.
- Chris Morley, MS Senior Human Factors Engineer, MAURO Usability Science
- Hannah Kang, MS Human Factors Engineer, MAURO Usability Science
- Elexa St. John-Saaltink, PhD, Research Scientist, MAURO Usability Science