Demystifying The Nuances of AI & ML For Your Regulated Medical Product
By Tim Bowe, CEO, Full Spectrum
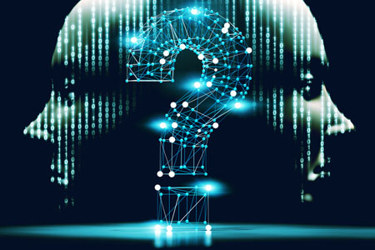
In a recent study, an AI-powered diagnostic system outperformed human doctors in accurately detecting breast cancer from mammograms. The AI achieved an impressive accuracy rate of 94.5%, while human radiologists achieved an average accuracy rate of 88.2%. This startling result highlights the potential of AI to significantly improve medical diagnoses and outcomes. The study demonstrates how AI algorithms can analyze medical images with high precision and potentially assist healthcare professionals in making more accurate and timely diagnoses, ultimately saving lives, and improving patient care.
Full disclosure: the claim above came from ChatGPT after a long dialog about AI in the medical space.
Initially, when we asked how AI will improve the medical field, ChatGPT gave the expected human-like responses about improvements in diagnostics, catching errors in product development, and enhancing treatment. It was all very agreeable and seemed as though it were a long-lost colleague. As we pressed further, ChatGPT was willing to be bolder with its dialog and claims, leading to the first paragraph of this article. What’s most illuminating is that when asked what resources were used in reporting that data, it couldn’t cite any sources nor back up any of its claims (as is appropriately called out in ChatGPT’s disclaimers). ChatGPT isn’t a replacement for engineers, and it’s certainly not currently built to provide medical advice. ChatGPT is what is known as a large language model (LLM, a special type of artificial intelligence [AI] neural network). An LLM takes in an enormous amount of training data (in this case documents written by humans) and learns human language patterns, digests what it believes are facts (true or not), and, when given a prompt, provides a response by putting together words and sentences to form what has the appearance of original thought, though, really, it’s closer to an advanced text generator. In this way, we set ChatGPT up for failure (and ourselves if we had really trusted it) by asking for data and insights for which it has no real-world basis or qualified expertise to provide.
Conversing with ChatGPT on the topic of AI in medical devices provides some insight into the challenges of integrating AI into a product with safety risks. A poorly formed question results in an equally poor response. In a medical device, how do you ensure that a poorly formed question does not result in a patient being harmed or misdiagnosed? Preventing patient harm or a misdiagnosis is something that medical device designers obsess about, and with the introduction of AI into medical devices, even more so. Medical device manufacturers can have the best chance of success in integrating AI by learning to converse within the AI landscape and selecting the correct AI architecture that fits their products and their patients’ needs.
Understanding AI And Machine Learning
There exists an ever-growing taxonomy in the field of AI and understanding all its nuances and branches isn’t just an exercise in vocabulary: it is paramount in deciding if AI is right for your business and end users.
The two buzzwords you are likely most familiar with are AI and machine learning (ML). They are often used interchangeably, but they mean two distinctly different things. Think of AI as the superset of a plethora of subtopics, which include ML. ML is focused on building adaptive algorithms that change internal parameters based on training data to adjust model fit (accuracy). Whereas AI leverages ML, instead of providing a deterministic output based on a known input, it’s built for evolution, adaptation, and enhancing beyond the initial ML inputs-outputs to provide an intelligent system.
Think of it like this: ML is to AI as your heart is to your circulatory system. Your heart is critical (arguably the most critical), but a heart without veins, arteries, or blood isn’t of much use. It’s in this way that your circulatory system is greater than the sum of its parts. Much is the same with ML and AI: ML alone isn’t AI, and AI isn’t much without ML components. When you take ML outputs and piece them together with other intelligence-building efforts, AI can emerge.
At the highest levels of AI, there are two main subcategories that will drive your architecture and, most importantly, your clinical outcomes: adaptive AI and generative AI. Adaptive AI is a type of AI that can automatically adjust to changing conditions and environments. It can interpret data and determine the best response or action. Generative AI is an AI system that can create new content or data based on existing data and patterns. Both adaptive and generative AI models have a place in medical devices. For something like ChatGPT, you may use adaptive AI – piecing together known bits of data to formulate a cohesive output. For creating personalized treatment protocols for patients, you may use a generative AI model.
Types Of AI Architectures Worth Considering
We asked ChatGPT if it could provide an exhaustive list of AI neural network (NN) architectures and it gave us an impressive 59 distinct well-known architectures, with an advisement that there are likely more due to rapid development of AI (note: the list it gave included errors we had to filter out, such as duplicates). There isn’t one AI architecture that fits every business, product, and technical objective. Learning the vocabulary to converse with experts is the first step. When researching whether AI is right for your business, consider these six popular AI NN architectures as being critical for foundational understanding:
- transformer neural network (note: the GPT in ChatGPT stands for generative pre-trained transformer)
- recurrent neural network (RNN)
- associative neural network (ANN)
- modular neural network (MNN)
- radial basis function neural network (RBFNN)
- feedforward neural network (FNN)
Selecting the right AI architecture will ensure you have a successful deployment that is risk-managed as well as safe and capable of providing the value-add to your stakeholders as well as getting through any regulatory bodies, such as the FDA.
Having a basic understanding of each AI architecture will better inform you of each architecture’s training requirements, limitations in its capabilities, and the risk it might impose on your end users. All of these will require trained development engineers, qualified testers, well-formulated test plans, and the usual truckload of documentation for the regulators.
AI’s Impact on Medical Devices (Current Vs. Future)
In the short time that AI has been available for medical device development, we’ve already seen tremendous adoption and shifts in its reception. Certainly, it has been marred by a mixture of fear and resistance, but it has also seen a significant counterpoint in its ability to provide diagnostic services, such as the identification of artifacts – including non-cancerous and precancerous (among others) companion diagnostic support information, advanced drug development, and Big Data analysis for risk factors and genetic analysis (Stanford study, 2022).
There are several themes that remain constant throughout the medical and AI community that will likely remain present in the near and distant future:
- How do I get my AI medical device through the FDA, or any other regulatory body for that matter?
- How do we manage change to the combination of the algorithm and the training set (a topic on which the FDA has recently released a draft guidance)?
- How does your training data fit with your data privacy strategy? (e.g., right to be forgotten)
- Does your training data match your target patient demographic?
- How do we adequately test the boundaries, manage risks, and ensure sufficient safeguards are in place to prevent an AI from causing patient harm?
While these questions, among others, seem to be common among medical device developers considering engaging with AI, the answers are not clear in all cases. Because AI is in its infancy, it will be quite some time before it feels normal to consider a pathway for AI in our medical devices. What’s important at this stage is that we take a page from AI, and we continue to educate ourselves, adapt to the changing marketplace and medical device landscape, and encourage our engineering and business teams to consider the aspects of AI product development that are different from traditional product development. Leave no topic unevaluated, including the following, when it comes to holistically evaluating how you plan to leverage the enormous power of AI in your medical device:
- access to (large amounts of) relevant, quality training data
- safety measures and testing procedures to fully verify and validate the AI model
- desired diagnostic or acceptable treatment ranges and outcomes
- AI regulatory guidance and its impact on associated submissions
- observability of your AI in the field
Current news articles often report AI’s ability to spot early signs of cancer or aberrant conditions (almost purely diagnostic). With the latest round of FDA regulatory guidance (FDA Draft Guidance on AI; April 3, 2023) and every tech sector abuzz with excitement over AI’s practical use in the medical field, you are likely to see a lot more of the same: AI used in an augmented, consultative fashion that allows the clinician to carefully evaluate the accuracy and validity of the AI’s outputs. Beyond this immediacy is the next AI frontier: AI for treatment. Think of the AI field as a small child right now. Currently, it’s observing and providing commentary from the backseat of the car while we drive. But, as AI grows up (as does our understanding of its utilization and capabilities), we’ll invite it to take the wheel and start to drive. So, rather than providing just diagnostic capabilities it might optionally provide treatment or interactive consultation. Examples of this include operating surgical robots, performing both invasive and non-invasive procedures, or augmenting medical staff for outpatient engagements where AI is more hands-on.
It should also be noted that combining AI with genetics and drug manufacturing may also lead to an upsurge in personalized treatments and medicines. While this is arguably the most exciting, it’s also an entire conversation on its own.
What we are unlikely to see anytime soon is truly unsupervised AI medical devices and AI autonomous robots from science fiction that make clinical determinations without their human counterpart. A practical example of this would be AI writing a prescription for antibiotics because a patient complained of a sore throat – a referral to a qualified physician, yes. But don’t expect Dr. ChatGPT to write you a prescription anytime soon.
Closing Thoughts
It’s true that AI is a burgeoning topic of aspirational engineering in almost all tech sectors. With AI comes a host of old and new challenges that every team will need to thoughtfully consider. Talking about AI and leveraging AI are two very different, but equally important, activities that need to continue to occur. Both require mastery of the AI field, intimacy with medical device development, and the ability to navigate the regulatory process to safely build AI into your medical products. If you are interested in building AI into your medical devices, for diagnostic or treatment, consider the entire spectrum of your product development, staffing it with experienced product development and AI engineers, and managing an ever-moving target of regulatory and market asks.
About The Author:
Tim is CEO at Full Spectrum. He has been an executive in the outsourced product development and engineering services industry for nearly 30 years, with domain experience and extensive writing in the areas of medical devices, healthcare information systems, automation, and robotics within complex capital equipment, as well as the development of products within the aerospace and telecom industries. Previously, he was COO of Emphysis and CEO of Foliage. Bowe has been an advisor and consultant to OEMs, engineering service providers, and the investment community.