Eye Tracking For Better User Interfaces
By Larry S. McGrath, Design Science
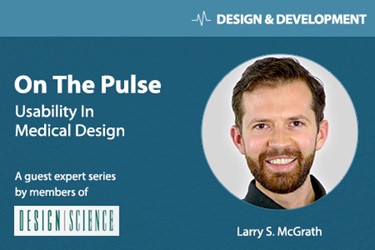
Advancements in eye-tracking technologies have made them convenient and affordable to incorporate into user research. Peering into an observer’s perspective can be a valuable source of data for optimizing diverse user interfaces. Few barriers remain to using eye trackers. The only question is how to do so effectively.
The expansive suite of tools that now come with commercial eye trackers may seem daunting to navigate – and time-consuming to learn. In truth, companies can develop basic competencies for eye tracking while simultaneously conducting fruitful research sessions. In this article, I offer some suggestions for gradually building up eye-tracking methods, in stages, to generate informative user data.
Whether it’s for a graphical user interface (GUI), instructions for use (IFU), or a website, the fundamental goal of eye tracking is to gather insights for optimizing the layout of visual information. Although the appeal may be to glimpse inside an observer’s perceptions, opening a first-person vantage point, eye trackers’ actual capacities are modest. They are one tool in researchers’ toolbox. Most notably, researchers can use eye-tracking video footage to complement the third-person observations collected from traditional user research. When brought together, perceptual, behavioral, and interview data cast different shades of light on the difficulties that emerge when testing a user interface. The combined data can provide crucial resources for design teams to create more intuitive, user-friendly products.
It’s worth mentioning that most commercial eye trackers accommodate only stable 2D surfaces. Although incredible strides have been made in 3D eye tracking, at present, it remains a frontier of user research.
Two kinds of eye-tracking technology are widely available: remote eye trackers and mobile eye trackers. The former is positioned on or near the object being tracked. Examples include the Tobii Pro Spectrum, SMI RED, and Gazepoint GP3. They’re useful to evaluate GUIs, since remote eye trackers can be affixed to monitors. Mobile eye trackers, worn on the head, include the Tobii Pro Glasses 2, Ergoneers’ Dikablis Glasses, and Pupil Labs’ Pupil Glasses. These mobile eye trackers have the advantage of affording a wider breadth of head movement, and are better suited to testing sessions in which participants must turn away from an interface. For example, an IFU with directions for assembling a medical instrument, or an operating room visualization system with information to guide surgical procedures, both require that users move their heads away from the interface to interact with devices.
Most commercial eye trackers come with a variety of analytical tools that can be used to cull quantitative and qualitative information from video recordings. Do text or images capture observers’ gaze for longer periods of time? How long do users spend reading each step in a task flow, and in what order? What text or images do observers skip over? These are some of the questions that eye tracking metrics can answer.
Before posing questions, there are a couple prerequisites. First, the user interface must be made amenable to data collection. Typically, researchers divide a sample image of the interface into areas of interest. Each area corresponds to a distinct region where observers might direct their eyes.
Second, an auto-mapping program superimposes footage of observers’ gaze points (that is, discrete time stamps and x/y coordinates) over the sample. What results is a wide-reaching bank of data about each area of interest. Examples of categories for such data include fixation duration, visit count, average visit duration, and time to first fixation.
Selecting categories to analyze might initially seem like poking around in the dark. Even among experienced researchers, different categories’ relative utility continues to be a source of debate. But rather than dwell on the meaning and merits of different categories, I’d like to suggest that it’s worth keeping in mind two broad kinds of data:
- Temporal data correspond to the duration spent observing an area of interest.
- Sequential data indicate the order in which areas of interest are observed.
Both data sets yield useful insights. Since they are expressed in seconds, temporal data can be easily aggregated, averaged, and compared. This quantitative metric allows researchers to discern which elements of a user interface preoccupy observers. Sequential data come in the form of gaze plots (otherwise known as scanpaths). These are qualitative visualizations that map individual fixation points over the sample image. They allow researchers to trace observers’ progression across the entirety of an interface. Combining both kinds of data can bring the parts — as well as the whole — of a user interface into focus.
So, what do temporal and sequential data tell us? The answer rests on researchers’ interpretive abilities. It bears repeating: eye tracking is one tool in the toolbox, and eye-tracking data are only as valuable as the valid inferences we can draw.
Inferences could be drawn on the side of the observer or the interface. On the one hand, researchers may interpret temporal data to reveal how long an observer pays attention to an area of interest. Similarly, sequential data might demonstrate the trajectory of an observer’s perception. In both cases, researchers infer the perceptual strategies that people use from eye-tracking data. On the other hand, researchers could examine how the interface exerts an influence on observers. Temporal data might be interpreted to indicate the density or complexity of visual information. Observers tend to spend more time fixating on areas of interest that are more difficult to comprehend. Then again, certain areas may capture the gaze longer if they are pleasing to see. Sequential data also can be interpreted to evaluate whether visual cues successfully guide observers’ eyes to critical elements. Researchers make inferences back and forth between the observer and the interface.
Eye tracking is an inferential endeavor. As such, growing pains are common when companies initially incorporate eye tracking into design research. Developing testing sessions in stages offers a chance to build up competencies. Consider the following three research scenarios.
Scenario 1: Observer Exploration
An early and informative testing model is to track observers’ eyes as they freely peruse an interface. Which elements draw observers’ gazes? In what order do they scan areas of interest? Unprompted exploration offers answers to basic questions about the arrangement of visual elements on a user interface.
Beginning with exploratory testing sessions is especially useful because the set-up is minimal. Putting an observer in a room, giving the person eye trackers, and setting him or her in front of an interface will suffice. In fact, a moderator is not even necessary. This is called an “encoding task.”
After browsing the user interface, temporal data — such as the time to first fixation and the duration of fixations — will illuminate which areas of interest preoccupy the observer. Sequential data also are revealing. The fixation percentage of individual areas is a useful measure to determine the proportion of observers who fixate at least once on a given area. The data can serve to rank areas according to how inviting they are.
However, raw eye-tracking data can be difficult to interpret. Many researchers recognize that, in the early stages of testing sessions, it’s easier to draw inferences by means of comparison. One useful metric is inter-observer consistency. Researchers can evaluate how well an interface guides observers by comparing multiple heatmaps. Overlap among the areas illuminated on each map indicates that the visual information directs observers’ eyes to similar elements. When consistency among maps is minimal, the interface likely offers poor guidance.
Scenario 2: Task Completion
Adding tasks can broaden the value of eye-tracking testing sessions. When a moderator prompts observers to perform activities, the results offer insights into not just the layout of a user interface, but also into its informational content.
The moderator could ask observers to complete real-time exercises. Perhaps there is a GUI whose purpose is to display biofeedback; or there is an IFU, which directs users to assemble an insulin pump. At issue is whether the interface enables users to achieve its intended function.
By focusing on intentional tasks, researchers can control the inferences that they draw from eye tracking data. When observation is goal-oriented, fixations are more likely to align with perceptual acts of attention, rather than – for instance – curiosity or distraction. For example, longer visits to an area of interest during an exploration scenario may indicate the observer’s level of interest in that area. During task completion scenarios, though, longer visits likely indicate that the area is complex.
Moreover, task completion results offer behavioral and interview information against which eye-tracking data can be compared. Perhaps the observer does not find a button to display heart rate data. Or maybe the person can’t successfully attach a tube to an insulin pump. In either case, researchers can directly observe the areas of interest where observers fixate or do not fixate when committing task errors. The insights help design teams make elements of an interface noticeable, especially when critical tasks are at stake.
Scenario 3: Interface Comparison
The optimal research model is not to analyze eye-tracking data against a single design, but to compare multiple user interfaces. This approach enables researchers to evaluate the relative merits of concrete options. In companies fortunate enough to have an active design team, one that can produce multiple user interfaces quickly, putting the interfaces before observers’ eyes is the ideal means to obtain actionable insights.
Interface comparison can be built on the research models developed in the task-completion scenario. Holding tasks constant across research sessions ensures that the interface is the independent variable being evaluated. In addition, interfaces should be counterbalanced to constrain order effects. That is, researchers should make sure to protect against observers’ bias by presenting them with different series of interfaces. As a result, researchers can use a rich bank of behavioral, interview, and eye-tracking information to draw inferences about how to optimize a design.
Eye-tracking technologies have become an indispensable tool for conducting meaningful user research. They are a source of immense value for generating intuitive and effective user interfaces. Building up testing scenarios in stages is an opportunity for companies to experiment with, re-evaluate, and perfect their eye-tracking research competencies. It’s a great way to tackle training and information-gathering – and make the most of an eye tracking investment.
About The Author
Larry S. McGrath received his PhD from Johns Hopkins University, where he specialized in the history and philosophy of the human sciences. At Design Science, he leads ethnographic projects involving the observation and analysis of medical devices in contexts such as hospitals, clinics, and homes.