Harnessing Connectivity In Medical Device Design
By Jesse Knight, senior mechanical engineer, BlackHägen Design
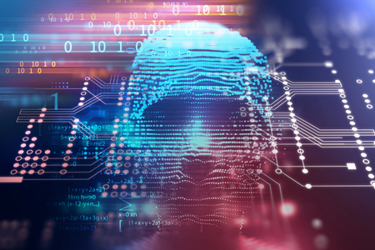
The current era of Big Data has transformed business, from baseball to advertising, and it has made deep inroads into medicine. There are still many gains to be made; adding some “smarts” to your next device is probably appealing. Medical device teams are working to collect, sort, and make sense of a ton of patient data, all with the goal of enabling better decision-making to improve patient outcomes. “Systems medicine,” where we are better able to leverage data from a variety of sources to make decisions, should drive us to provide care that is predictive, preventive, personalized, and participatory. Creating large data sets also creates new opportunities for public health and research. This article will discuss some of the thought processes that can be helpful when designing a connected device.
When we add features to any device, we need to ask ourselves: what am I improving for the patient? Does this allow new kinds of treatment? Are we offering them more accurate care? Does it make their life more convenient? That convenience can mean someone is more likely to seek healthcare or complete a course of treatment successfully. Being intentional about what benefits we are providing both patients and providers helps keep the device design process more focused and manageable.
Assessing The Value Of Network Connections
Connecting a device to a network adds value where the sum can be greater than the parts. Continuous monitoring provides a higher level of vigilance than a clinician and helps shift care from reactive/episodic visits to proactive/preventive medicine. Combining data from several sources can offer more context during analysis, with correlation allowing a better total picture of what is happening during the patient continuum. Closed systems that isolate data are less useful to clinicians, but ecosystem lock-in may be attractive to companies. Consider formatting your data to a standard like Fast Healthcare Interoperability Resource (FHIR) so that the decision on when and how to share data is one of permissions and not one of technology.
Unfortunately, putting a datalogger in a device and connecting it to a networked database is not a panacea. You’ll need to consider the trade-offs. First, you’ll need to solve the structural issues: storage space and duration, security vs. ease-of-access debates, translation for other systems, how to correlate the data with that collected from other devices, and so on. Then, you’ll need to look at larger issues. For example, is the data you’re collecting believable? Useful? Are you creating a privacy concern by over-collecting? Can this data be misused? Are you oversampling, which may lead to more testing and possible overdiagnosis/overtreatment?
How To Make The Most Of Your Data
To address the question “how can we make the most of this data?” many companies are turning to artificial intelligence (AI). A number of conference speakers, startups, trade articles, and every third LinkedIn post seem to imply that AI will solve all of our problems (and possibly lead to the end of civilization). It’s still too early to call it a revolution, but it looks like this shift will have more legs than blockchain or other topics that have captured so much attention.
In this conversation, “AI” refers to any kind of “thinking machine,” whether it’s neural networks, machine learning, large language models, or something more generative. In the context of medical devices, AI is used colloquially to refer to anything that is making decisions in the machine rather than referring the data to a clinician.
In the absence of hospital review boards, medical licenses, courts, and the other means we use to hold humans accountable, we must judge the decisions coming from software. In other words, how do we improve those decisions? Much of that comes down to how the software is trained.
A good training model will be strong in three areas:
- Quality: There is no absolute standard for measuring the quality of decisions that a computer makes, but the absolute minimum should be consistently as good or better than humans.
- Diversity: A strong AI model picks up on less common patterns in the training data without degrading the outputs. This helps reduce bias in the models and can help find patients with faint signs (early detection can make a huge difference in restoring health) or less common presentations. A good training model needs diverse input to learn from.
- Speed: The system must make decisions quickly enough to be clinically relevant. Some systems benefit from being very fast — adjusting a setting quickly enough to smooth out a ripple in a process — and others will just need to make sure patients and clinicians aren’t wasting their time waiting for a decision. Each of these provides an opportunity for more personalized and accurate care.
Once trained, a computer model can be deployed to make many decisions for many patients without incurring nearly the cost of having a human make each of those choices.
Looking To Regulatory Standards
There are risks associated with adding decision-making to a product as well. The FDA’s standards on measuring and monitoring have not been written to address the range of possibilities available to us today. Companies must choose whether to forge ahead and hope the regulatory bodies catch up in a way that doesn’t require altering their product, to sit back and wait for guidance, or to work in the margins around medical devices and not implement machine intelligence directly into 21 CFR 820 products.
Legal and regulatory hurdles are only the first step. Will hospitals and clinicians use our devices? Will patients? There is a gap in trust that we must overcome. Drivers seem split on whether they’ll embrace self-driving cars. Would you let one take your child to elementary school without you? Many wouldn’t. What does that say about using a device that affects my healthcare decisions in less obvious ways? We’re just beginning to learn the anxieties we’ll need to address as device designers. Some will resist because they fear being tracked. Some will be Luddites. There are new human factors concerns — some of which will be improved by new opportunities like voice control, but that will present new problems as well.
Of course, the biggest hurdle is ensuring your device makes good decisions. Can you tolerate an occasional malfunction or poor choice? There is no system for making perfect choices; humans certainly don’t do it now. Scenarios where there is no one correct answer, or the balance of trade-offs is one of personal taste, are common. How gracefully will the intelligence in your device “hand the steering wheel back to a driver”? Even software that works perfectly as intended all the time (not possible in a complex environment) will run into situations that are difficult or will be judged harshly after the fact. Society has created ways to address human shortcomings, but we’re still learning to address automated ones.
It’s up to device designers to navigate the pitfalls and produce devices that add value to healthcare. Improving decision-making to improve patient experience should always be the goal. Whether that means earlier engagement, more effective treatment, less disruptive care, or lower cost, keeping patient outcomes firmly in mind is the best tool we have to keep us on the right track. If you have any doubts about artificial intelligence, try to pick out which of these paragraphs was written by ChatGPT.
In Summary
There’s no doubt that the “systems medicine” approach that incorporates data gathered from a variety of sources will help drive new and improved methods of patient care. The creation of larger data sets over time will provide exceptional opportunities for a wide swath of those involved in healthcare — from private and public health administrators and researchers to clinicians and patients — and may even relieve some pressures on the healthcare delivery system itself.
However, to provide care that is predictive, preventive, personalized, and participatory, when considering the development of connected devices, make sure that the device demonstrates a clear clinical advantage, provides safety, will be accepted by the target markets (patient and clinical), and delivers an acceptable ROI.
According to Mordor Intelligence, the 2023 global market size for connected medical devices is valued at $57.48 billion. By 2028, the segment is expected to reach a value of $115.51 billion, a CAGR (2023 to 2028) of 14.98%. Clearly, this demonstrates significant opportunities for medical device designers and manufacturers to embrace new technologies. However, they must be selective when seeking partnerships in the design process that are not only current with regulatory guidelines but also will provide guidance to ensure clinical advantage and use safety and can help manage development costs to deliver profitable outcomes for all.
About The Author:
Jesse Knight is a senior mechanical engineer at BlackHägen Design working as part of a multi-disciplined team designing medical devices for both big and small companies. He earned his degree in mechanical engineering from Walla Walla University.