How To Optimize Reliability And Cost In Surgical Robotic Systems
By Chris Illman, PA Consulting
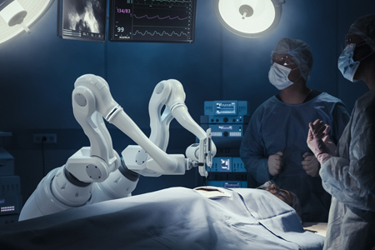
The tension between reliability and cost has never been higher in robotically assisted surgery (RAS). For systems in development now, a laser-focus on reliability is the route to success. These competing factors aren’t as antithetical as they seem, and the right approach can optimize for both simultaneously.
Early RAS systems designed and developed by Intuitive were cited as disrupting 2.5 percent of operations with an equipment failure that might have resulted in technical work on the machine or conversion to laparoscopic or open surgery — increasing patient risk. While 2.5 percent was not a huge number, it was a significant number and clearly needed to be minimized where patient outcomes could be compromised.
Nearly 25 years on, systems jostle for space in the operating room, competing not only on their own unique functionality or specialty but also on cost of purchase and ownership. Systems are expected to be both cheaper and more reliable than before. This presents a challenge for new players in the scramble to market.
Competing Challenges
Having a reliability team or dedicated reliability engineer is often viewed as a luxury even in established companies, not to mention startups. At worst, it can be seen as a hinderance or a stage gate to jump through at the end of development. In a world of funding rounds and aggressive timelines, reliability and cost are seldom prioritized for demonstration.
Prevalent attitudes lead to the same mistakes on repeat. Overengineering is seen as the free fix for reliability, but it doesn’t always help. The cost of goods (CoGs) increases, often alongside the part count. This is highly likely to make reliability worse.
The opposite mistake is to adopt a head-in-sand belief that reliability will just emerge during general development – particularly popular where cost targets are front of mind. Life testing (crucial for a product that stands the test of time) suffers a similar fate, with businesses glibly using their first adopters to test reliability. Releasing a product that isn’t ready can provide important feedback, but it can also poison the market against the product in perpetuity. A further challenge is the tendency to confuse perceived quality with reliability. Perceived quality is easy to create (e.g., with clever industrial design), while reliability requires conscious effort, expertise, and planning from the start.
Getting The Balance Right
With so many ways to get it wrong, how do we optimize for reliability and cost simultaneously? How do we ensure reliability without surgical robots becoming too restrictive, slow, and costly? How will it help to reduce CoGs?
First, know your acronyms — they’re often misrepresented:
- MTTF: Mean time to failure represents the time it takes for half of in-field machines to fail, often used interchangeably to describe reliability.
- MTBF: Mean time between failures is the time between fixable faults. This is more useful.
- MTTR: Mean time to repair is much more interesting for a complex system like a robot.
- MTBS: Mean time between service has a clear interdependency with MTBF.
- Availability: A combination of MTBF and MTTR, this is a measure of how often a system will be available, which is highly useful to the clinician. This is what we really need to optimize for.
- DFR: Design for reliability is the discipline that brings this all together.
Profiling a system for high availability is the art of balancing all of the above factors against CoGs and cost of development without demanding 100 percent reliability from the machine. “Best” is the enemy of “good enough” here. The graph below shows the sweet spot. The lowest total cost (considering development and cost of failures) appears at less than 100 percent reliability – good news. The total cost line is typically heavily skewed to the right, where further work on reliability has diminishing returns. To optimize for cost and quality, target “good,” not “perfect.”
Get The Right Management In Place
Creating a reliable product is a management task. Instate a dedicated DFR workstream early, with the leadership to make the engineering team accountable for driving the appropriate reliability balance. Do this no matter how small your design team is, and you’ll see the benefit in CoGs and cost of ownership. Here are the inputs:
- Establish a business directive – set the limit you’re willing to spend on repair and replacement when in market and the scope of a reliability program.
- Set high-level reliability requirements around availability and lifespan (MTBF).
- Be realistic about MTBS – you do want service touchpoints with a robot, and none will or should ever be free from that.
- Develop market insight – know what clinicians will expect and, crucially, how your equipment will be used. Only when the environment is known can you design for it. Not being the first robot to achieve clearance helps here (in fairness to Intuitive’s early da Vinci models).
- Let your reliability engineer carefully assess any standards and mandated reliability requirements; the BS5760 series is a great handbook for navigating the path, but particular standards may mandate reliability for safety.
- on’t double-count safety margin at specification – empower your reliability engineer do this to avoid driving up the CoGs.
An engineer with a reliability mindset should target reliability with low CoGs and operating cost at an early stage in the development project. Simple designs tend to be inherently reliable, low in cost, and come together quickly, but design teams have a habit of straying from that path without guidance.
Leverage Life Testing
The best predictor for in-field reliability at the R&D stage is a meaningful, representative life test — a long and scary line in the development GANTT chart and mandated by developing regulations. If the reliability workstream was successful, this should be plain sailing — a confirmatory check. Subsystem testing through development should leave no surprises, no expensive rework, and no sticky tape quick fixes prior to product release. The system will be neither too reliable nor unreliable, with appropriate service intervals and recoverable error states.
It takes considerable skill to accelerate life testing while still getting meaningful results. Surgical robots have an advantage over many other complex systems here as much of the loading is intrinsic. Factors such as self-weight, wire tension, and thermal management make up the lion’s share of service loading. These factors are predictable and can be used to streamline life testing successfully. Get this testing right, and you’ll go to market with the confidence that clinicians’ perceptions will be positive and there will be no costly recalls or warranty claims.
High availability at launch will help establish a reputation for excellent quality at a given price point. After product launch, continuous monitoring of reliability is an ongoing task. If the system is well characterized for reliability, it is prime for further cost-out. Ongoing monitoring will highlight areas for further optimization and build valuable data that may help extend MTBS or equipment life or highlight a further CoGs reduction. The natural extension of monitoring is real-time telemetry to monitor equipment usage and health, allowing predictive maintenance and repair, and even informing a smart supply chain. Closing that loop allows mastery of machine availability.
The tension between reliability and cost in surgical robotics has never been higher. But, by prioritizing a robust methodology for reliability engineering, you can get the balance right.
About The Authors:
Chris Illman is a biomedical engineer based at PA Consulting’s Global Innovation and Technology Centre in Cambridge, England. He has years of experience developing new products and bringing existing products into compliance.