How Will Advanced Computing Technologies Catapult Medtech Into The Future?
By Elliot Turrini and Ben Locwin
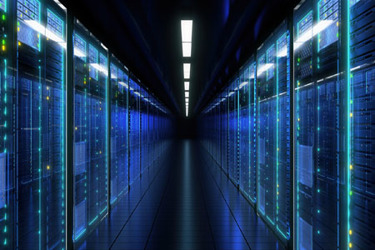
There is an almost unlimited profit potential for medtech manufacturers to use advanced computing technologies such as artificial intelligence (AI), faster and smaller computing hardware, computing-driven robotics, augmented/virtual reality, and Internet of Things (IoT) to build devices that disrupt markets and improve people’s lives (we term this collectively “digital medtech”).
As we noted in our last article, “three forces are combining to make it vital for industries [such as medtech]” to find ways to cost-effectively use advanced computing technologies:
- Huge profit opportunity from looming healthcare services shortages: Many experts predict a large shortage of physicians and nurses in the United States over the next decade, which provides life science companies the opportunity to use computing technologies to build very profitable medical devices, digital therapeutics (DTs), prescription digital therapeutics (PDTs), and healthcare services. The number of patients who are being treated — in part or in full — by digitized approaches is growing dramatically, and digital therapies are the only way to treat patients of the future, given healthcare's rising resource shortages.
- Substantial competitive pressure: Today's business world is in the middle of a computing technology revolution, which is creating immense pressure to use increasingly sophisticated computing technologies (like AI and IoT) to innovate offerings and operations.
- Healthcare services are primed for disruptive innovation: The late Harvard Business School Professor Clayton Christensen, in his book Innovator's Prescription, explained at great length how the healthcare industry has been slow to adopt new technologies, leaving it vulnerable to (and in need of) disruptive innovation — particularly by harnessing sophisticated computing technologies.
Because new digital medtech can improve the efficacy and outcomes of existing interventions and services, reduce their costs, and/or perform new interventions and services, they open almost unlimited profit opportunities for device manufacturers. This is why so many companies are rushing to build digital medtech such as those we’ll describe here.
To help medtech manufacturers identify ways to profitably harness advanced computing technologies, we identified three main functional advantages to explore:
- Using data analytics (including AI) to improve the efficacy/outcomes and reduce the costs of existing interventions: e.g., a digital stethoscope.
- Making more effective implantable devices: e.g., an artificial heart that harnesses computing technology.
- Collecting new forms of patient data or finding new ways to analyze existing data that open new data analytic opportunities and/or new interventions and services: e.g., using machine learning algorithms to predict disease evolution and treatment outcomes.
The rest of this article explores each main functional advantage and it ends with a vital warning about the almost unlimited potential liability from a device manufacturer’s failure to properly secure digital medtech.
Using Data Analytics And AI To Improve Existing Interventions
This category is the most common way that medtech manufacturers are leveraging advanced computing technologies. Consider these many examples:
Eko: It created and manufactures digital stethoscopes that use amplification, noise reduction, algorithms, Bluetooth connectivity, and a mobile app to better screen for heart conditions such as heart murmurs and atrial fibrillation.
NXgenPort: It is adding remote monitoring of chemotherapy patients with implanted chemo port catheters, with the goal of reducing the incidence of related infections. It is also developing microelectronic sensors to monitor physiological data during cancer treatment, such as blood cell counts, heart functions, oxygen levels, body temperature, device leakage, and more, according to the company.
Prenosis: It is developing an "assisted intelligence" system to better diagnose sepsis (historically very tricky), using a machine learning algorithm and holistic input data from 23 parameters including three biomarkers (Interleukin-6, procalcitonin, and C-reactive protein) to help profile the patient's underlying biological state accurately.
Spark Biomedical: It created and sells an FDA-cleared, drug-free, wearable technology – the Sparrow Therapy System – worn around the ear for opioid withdrawal relief. The system has three parts: (1) an earpiece that delivers mild electrical stimulation to the nerves around the ear to release endorphins and reduce symptoms; (2) a Patient Controller that adjusts the intensity of the electrical stimulation; and (3) a Clinician Programming App with Bluetooth that improves how clinicians can treat patients.
In this category, the most used computing technology is some form of data analytics that delivers better, more actionable insights that can improve the efficacy of existing interventions and/or reduce their costs.
The use of data analytics can be a very powerful way to improve the efficacy of existing interventions and services, such as Eko’s use of a digital stethoscope that leverages algorithms and related technologies to improve the efficacy of the traditional use of stethoscopes: (1) it improves the sound quality (e.g., noise reduction and signal amplification) and (2) facilitates data analysis and sharing via its mobile app and dashboard. Another good example is NXgenPort’s combined use of microelectronic sensors and data analytics to provide actionable insights in real time to healthcare providers, which empowers them to improve their cancer treatments.
Data analytics offers excellent profit opportunities and is – from an important perspective – less complex and hence at times easier to implement than the next category – making effective implantable devices.
As an important side note, companies using data analytics will eventually have to account for the FDA’s Data Modernization Action Plan.
Making More Effective Implantable Devices
This category is growing – and it might include the most difficult of the computing and engineering challenges – and its growth will accelerate as more companies like the three summarized below succeed in their ventures:
Synchron: It has developed a brain implant, “brain.io,” that it calls a “motorneuroprothesis,” which is designed to bypass “damaged neural pathways in patients with severe paralysis” in ways that help restore motor capabilities so the patient can control digital devices. Brain.io has three parts: (1) an “endovascular implant, placed in a vein alongside the motor cortex, which generates any signal related to movement”; (2) a “receiver device implanted in the chest [that] transmits the neural signals to a decoder”; and (3) a “machine learning algorithm [that] translates those signals into specific digital commands.”
BiVACOR: It has developed “a total artificial heart designed to take over the complete function of a patient’s failing heart.” It has two parts: (1) the implantable computer-based mechanical heart that replaces the heart’s pumping function and (2) an external controller with battery power.
Onward Medical: It has developed “ARC Therapy,” which uses proprietary software and either an implantable (ARCIM) or external (ARCEX) system to deliver targeted, programmed spinal cord stimulation that helps restore movement and other functions in people with spinal cord injuries. ARCIM comprises an implantable pulse generator and lead placed near the spinal cord, which is controlled by wearable components and a smart watch that runs Onward’s proprietary software.
The companies in this category will typically combine computing hardware, firmware, software, different forms of data analytics, and connectivity between the implanted devices and external computing technologies.
Synchron, as mentioned above, is an excellent example. It created a system to restore some motor capabilities to patients with severe paralysis, which includes two implants, machine learning algorithms, and connectivity to two external computing devices. BiVACOR’s total artificial heart represents an astounding use of computing technologies and other engineering advances. It performs the blood pumping function of the human heart by an implant that uses a microprocessor electromagnetic field that is connected to an external computing-based controller with battery power. These companies are just two examples of companies in this category, and many more similar device companies like these will be created in the foreseeable future.
Using Data Analytics To Develop New Opportunities And/or New Interventions And Services
There is almost limitless potential for medtech to find new interventions and services by using AI to analyze existing data and/or by developing technologies that identify previously unknown aspects of human physiology and convert those insights into actionable data. Consider the radical improvements in healthcare gleaned from the mapping of the human genome – which at the time was a previously untapped aspect of human physiology.
Medtech’s combined use of (1) new devices that gather new information about human physiology and health and (2) AI to better analyze and leverage this new information and existing patient data presents a great opportunity for medtech manufacturers. This category, therefore, might offer the greatest opportunity to disrupt markets because it enables medtech manufacturers to discover previously unknown interventions and services.
Consider these three examples:
BioSymetrics: To improve the drug discovery process and aid in the development of precision medicines, BioSymetrics uses a three-step process that: (1) gathers diverse clinical and experimental data from large hospital systems, pharma companies, and genomics partners; (2) uses its proprietary machine leaning – called Contingent AI – to analyze the data and produce actionable insights; and (3) leverages phenotypic animal models for experimental validation. This process is a new way to analyze existing data that creates opportunities to develop new powerful drugs and interventions.
Owkin: It combines life science and machine learning expertise to make drug development and clinical trial design more targeted and more cost effective. Owkin's machine learning algorithms create models that predict disease evolution and treatment outcomes. These predictive models are used for enhanced analysis, surrogate endpoints, patient stratification and selection, and subgroup identification. Its approach speeds up drug discovery and lowers the costs of creating new, better treatments.
Aptar Pharma: It uses integrated digital solutions that leverage AI to help enhance patient outcomes – e.g., improve the management of the side effects of cancer and immunology treatments. Aptar supports pharmaceutical companies by leveraging a combination of real-time patient data, software, AI, and hardware platforms to deliver more personalized digital health interventions to patients and their care teams. These end-to-end interventions take shape as digital therapeutics that at times are combined with connected drug delivery devices (e.g., a smart inhaler).
Aptar’s model of applying AI to patient-level data in real time yields valuable insights that can enhance patient outcomes – particularly when combined with connected drug delivery devices. “Our clinical algorithms engine is the heart of our digital interventions [and what usually determines their status as software as medical devices],” said Pierre Leurent, president, digital health, Aptar Pharma. “Embracing AI, and data analytics in general, enables us to better understand patient care trajectories and continuously refine our interventions to make them even more personalized and impactful. In addition to helping patients enjoy a better treatment experience, digital therapeutics provide a very valuable new source of real-world evidence and actionable insights to optimize care delivery and enable value-based care.”
Practical Tips For Using AI In Medtech
The term artificial intelligence (AI) describes how algorithms can be used for tasks and decisions that emulate human intelligence. While AI opens to medtech manufacturers amazing opportunities, it comes with material risks such as inaccurate data, problems in how the data is trained, adversarial attacks on the AI, privacy violations, and of lack of “transparency” of the overall process. A good resource to help medtech use AI and machine learning are the 10 guiding principles for Good Machine Learning Practice for Medical Device Development, issued by the FDA, Health Canada, and the U.K.’s Medicines and Healthcare products Regulatory Agency. The first three principles are:
- Multi-Disciplinary Expertise Is Leveraged Throughout the Total Product Life Cycle: This recommends staffing AI projects with the right mix of technological experts and healthcare professionals who fully understand the desired results, such as improved patient outcomes.
- Good Software Engineering and Security Practices Are Implemented: This means applying “good software engineering practices, data quality assurance, data management, and robust cybersecurity practices.” For example, this includes using “methodical risk management and design process that can appropriately capture and communicate design, implementation, and risk management decisions and rationale, as well as ensure data authenticity and integrity.”
- Clinical Study Participants and Data Sets Are Representative of the Intended Patient Population: This recommends that as to your data, take steps to “manage any bias, promote appropriate and generalizable performance across the intended patient population, assess usability, and identify circumstances where the model may underperform.”
Based on our discussions with companies and people who have successfully implemented AI, medtech companies should also consider the following:
- Extra time: Compared to traditional software projects, AI projects typically require more time and up-front effort – particularly in the planning stage.
- Leverage open-source: Many AI projects can reduce costs by leveraging open-source technologies such as TensorFlow and Apache SystemDS.
Special Warning About Liability From Cyberattacks
The next article in this series will detail how this unlimited profit potential comes with almost unlimited liability from cyberattacks. To set the stage for the next article, consider the March 2, 2022, report from Unit 42 showing that it found known cybersecurity vulnerabilities in 75% of the over 200,000 infusion pumps it analyzed. Vulnerabilities like those are exactly the types of gaps that cyber-attackers can exploit. The next article provides information about all-too-real and scary cyber-incident scenarios and how to cost-effectively mitigate them.
About The Authors:
Elliot Turrini is the CEO of Practical Cyber, a cybersecurity and privacy firm with a medtech specialty. He has been a federal cybercrime prosecutor, a cyber/privacy lawyer, and digital health entrepreneur. He is the co-editor of the book Cybercrimes: A Multidisciplinary Analysis.
Ben Locwin is a healthcare futurist and medtech executive, working to bring the future of better healthcare technology within reach of all patients in need. He has provided oversight to trials of COVID-19 treatments and top vaccine candidates and participates as a member of several state and federal public health task forces. He has spent the last few years working on solutions for cybersecurity issues impinging on medical device regulatory approvals and safe commercial use.