4 Lessons For AI In Medtech: Case Studies From Breast Cancer Detection
By Andrew Smith, Ph.D., vice president of image research for Hologic, Inc.
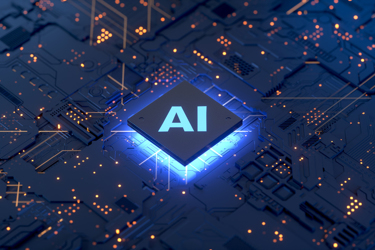
The detection and prevention of breast cancer has come a long way in the past 20 years, thanks to advances in digital mammography and 3D mammography. Radiology diagnostic performance is improving, death rates are declining, and more individuals in the United States and around the world are getting access to state-of-the-art screenings. Now, recent advances in machine learning, especially deep learning, are poised to help further advance the detection and diagnosis of breast cancer.
As one of the physicists involved in helping advance breast health solutions through this inspiring artificial intelligence (AI) evolution, I’m at once very pleased with our progress and acutely aware of how we got here and how much further we will go. Across the medical industry, research and development teams are working diligently to bring new AI-fueled innovations to life. However, as promising as this advancing technology is for bringing greater certainty and peace of mind to the patients and healthcare professionals who care for them, many challenges must be overcome, especially as regulators wrestle with how to assess these increasingly complex technologies.
Leveraging the development of the mammography technology, this article shares lessons learned and key considerations for successfully bringing the AI medical devices reliably to life – from concept through development, approval, launch, and adoption.
Quick Overview Of Referenced Technologies
Before jumping right in, let’s define our terms. For reference:
- AI is a broad term used to describe machines or computers that mimic functions of human cognition.
- Machine learning (ML) technology is a subset of AI. It uses statistical models that can be trained using known data samples to perform a task at hand, such as detection of specified features from images.
- Deep learning (DL) is the next generation of machine learning. It uses the massive computational power offered by graphical processing units (GPUs) to train very complex statistical models that contain hundreds of layers of parameters and are therefore referred to as “deep.”
Lesson 1: Avoid Unintended Consequences
In screening, trained radiologists read mammogram images of the general population to identify potential abnormalities. Even the best performing radiologists find some cancers, miss some cancers, and falsely identify non-cancers. Typically, a radiologist reviews four digital image sets per individual, two per breast, on a computer screen. While this takes time and produces some errors, it is a vast improvement in efficiency and accuracy over the old days of relying on manual exams and/or reviewing X-ray films on light boxes. The latest technology, 3D mammography, requires the radiologist to review hundreds of images per individual.
About 20 years ago, radiologists began using computer-aided detection (CAD) as a virtual second opinion for breast cancer screenings.
The original CAD algorithms worked by searching mammograms for characteristics of breast cancer. AI scientists programmed software to detect these “features,” and then modified and trained the algorithms to maximize their performance. In clinical use, the CAD system scans mammogram images, searching for and marking potential abnormalities for human review. This breakthrough technology was designed to improve detection accuracy by helping radiologists avoid overlooking pathologies.
It went through clinical trials and studies, gained regulatory approval, and quickly found its way into the real world. So far, so good.
Eventually, however, clinicians in the field identified limitations. One limitation was the CAD algorithm missed many cancers. And more importantly, in the real world, where only about five in every 1,000 U.S. women has cancer that can be detected using mammograms, the algorithm generated marks on nearly every study, necessitating review of each of the “false” marks, so as to avoid missing cancers.
This was a significant lesson learned. That product aimed to help radiologists avoid missing a cancer they may have overlooked. In the real world, it probably did make a small positive difference in cancer detection, but it certainly also increased the radiologist’s workload in reviewing and dismissing all the false marks.
It is important to be super diligent in thinking through all the implications of an AI product, including its initial purpose and all the ways the product might change users’ behavior.
Lesson 2: Recognize That AI Algorithms Work Best Using Objective Truth
The next tough lesson comes from a product developed to measure breast density. For reference, breast density is the amount of fibrous and glandular (heavier) tissue compared with the amount of fatty (lighter) tissue. In mammography, this is important for two main reasons: 1) dense breasts have a higher risk of getting breast cancer, and 2) mammograms of dense breasts are less accurate. In the U.S. radiologists routinely report on breast density and, in some states, clinicians must notify patients found to have high density breasts because of the risks cited above. With this purpose, an algorithm was programmed to read a mammogram and provide an assessment of breast density. These original algorithms were determining objective breast density for which an algorithmic approach was available, so AI was not needed in this initial product.
However, once in use on market, some radiologists objected, because the product, even though calculating breast density in a “true” manner, often disagreed with their subjective assessments. Additionally, during this time, the actual clinical definition of breast density that a radiologist would use changed to a more subjective measure. State-of-the-art breast density algorithms use machine learning, not to more accurately determine actual breast density, but rather to more closely mimic how a radiologist will assess breast density, regardless of the “truth” of the radiologist’s assessment.
Machine learning requires objectively factual data be input in order for the algorithm to learn what the truth is and to train to identify it. However, with breast density, the quality of the training data is not precise.
Therein lies the lesson. Breast density is a subjective measure based on the radiologist’s assessment – no single truth exists. In fact, oftentimes two radiologists reviewing the same mammogram will rate breast density differently (known as inter-reader variability), just as the same radiologist might if some time has lapsed between assessments of the same mammogram (known as intra-reader variability). The lack of established real truth of breast density is a challenging application for AI. How can one design an algorithm that will agree with all radiologists if they don’t agree amongst themselves?
Lesson 3: Define The Question You Want To Answer, & Don’t Be Afraid To Pivot In Your End Goals
There are several areas where clinical performance in mammography can be improved. One is improving cancer detection by helping radiologists avoid missing cancers. This was the goal for the original CAD product. Another goal is to help reduce false positives, which limits unnecessary additional imaging and reduces healthcare costs. Yet another is to address the radiologist’s workload, which has only become worse with the introduction of 3D mammography and its hundreds of images. Each of these are useful goals for an AI product.
For example, if the goal is breast cancer detection, then the AI must learn to identify all signs of potential abnormalities, even faint ones. The trade-off is the AI likely also generates more false positives. But this may be a trade-off that customers are willing and able to make.
Consider another example where we want to reduce false positives. In the United States, to find the on average five cancers per 1,000 women, a full 10%, or 100 women are called in for follow-up exams, and being called back in often causes emotional stress to the patient, as well as financial strain (e.g., taking work off, arranging for childcare, paying deductible, etc.) and physical discomfort. It also increases the total cost of care. If AI can help reduce a fraction of these false positives up front, it can improve healthcare and reduce costs. However, unless the AI is totally accurate, the risk is reducing false positives might also mean that, hopefully rarely, a cancer is overlooked. But this eventuality needs to be considered and weighed.
If the population of 1,000 women only has five cancers, then 995 mammograms do not show disease. Can we use AI to find these? If AI can help identify even a fraction of these disease-free mammograms, then potentially these mammograms will not need to be carefully read by radiologists, as they are today. In such a scenario, the physician’s workload is reduced without adversely affecting patient care.
In these three examples, regardless of the problem you aim to solve, the AI is trained on the same data, i.e., verified mammogram images, but programmed differently. For the reasons described above, each algorithm is created to serve a specific purpose in solving a stated problem. But each successfully reached AI goal carries trade-offs.
The takeaway here for product developers is to know the questions you can train AI to answer and anticipate and understand the trade-offs whenever it does. That way, you can consider whether and how to address them with additional algorithms or product innovations. For example, in lesson #2 above, the product proved of value for another purpose. Every time the algorithm assessed a mammogram for breast density, it generated the same ratings. Its human counterparts did not. Hence, the AI offered clinical settings consistency in care, and this became one of the product’s main selling points. Perhaps, in retrospect, this should have been the goal in the first place, rather than asserting that the algorithm determined real “truth” when no such truth existed.
Lesson 4: Moving Forward, Be Prepared For “Show,” Not “Tell”
Knowing the main role of a regulatory body is to assess the safety and effectiveness of the product under review, you need to be able to explain how your product works and answer regulators’ detailed questions related to efficacy and safety. As with the full spectrum of medical products, the better you can articulate how your AI works and why, the more comfortable regulators will be.
Machine learning-based AI algorithms are easy to explain, because they are trained in the same manner as a human would be: “If I’m a radiologist who is an expert in reading mammograms and I have a new resident who wants to read them, I’d show her what breast cancer signs look like and what false positives look like, and teach her what to look for. For example, breasts are usually symmetric, if you see something on one and not the other, that’s suspicious. If you see a round mass that has irregular borders, that’s suspicious…” So, for ML algorithms, we can state that the algorithm searches for rounded masses with irregular borders, and it searches for asymmetries in breasts, and so on.
With deep learning (DL), however, explaining how the algorithm works is easier said than done, because it uses a completely different method of training and programming. With DL, the AI is given a comprehensive series of mammograms and trains itself. The model is not trained to directly look for features of cancers as was done with conventional ML algorithms. How it succeeds, what it is flagging, no one knows. Also, nobody knows under what circumstances the algorithm will make mistakes. This technological riddle makes regulators less comfortable during review and approval processes. The upside is DL AI is showing superior performance to older ML AI, and so this is well worth pursuing.
Still, DL-driven medical products recently have been approved, including the early breast cancer detection medical technology that finds cancers with improved accuracy. DL solutions also are showing promise in being able to identify mammograms that are clearly benign. So, a precedent for approval is being set, which is, while you cannot explain the inexplicable, you can demonstrate performance through tests and studies. This brings us to our final lesson learned: For the most advanced AI products in development, plan ahead in terms of program management and resources to do more showing than telling.
About The Author:
Andrew Smith, Ph.D., is the vice president of image research for Hologic, Inc. He has been involved in medical imaging research and development for over 30 years, with his current focus on advanced breast imaging technologies such as 3D tomosynthesis. He lectures widely around the world on breast imaging and is a named author on over 100 U.S. patents. Prior to Hologic, he co-founded Digital Scintigraphics, a company that developed high-resolution nuclear medicine neuroimaging systems. He received his B.S. and Ph.D. degrees in physics from MIT in Cambridge, MA.