Managing Contamination Risks In The Pharmaceutical And Medical Device Industries Using Relational Risk Analysis
By Mark F. Witcher, Ph.D., biopharma operations subject matter expert
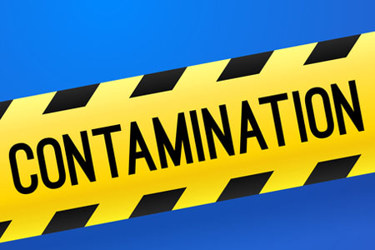
Of all the risks associated with pharmaceuticals and medical devices, controlling contamination is one of the most important and difficult challenges. Currently, contamination risks are largely managed by compliance with guidelines such as EU GMP Annex 1 and ISO 14971.1,2 This article describes how relational risk analysis (ReRA) models can be used to understand and analyze contamination risks for efficiently building risk-based contamination control strategies (CCSs).
A risk analysis approach that describes, analyzes, manages, and efficiently communicates a specific contamination risk is more effective than managing the risk by compliance to a generalized, one-size-fits-all guidance. The best approach to building a CCS is to define and design it using a ReRA risk-based approach and then check it for compliance prior to executing the qualification and verification stages of the CCS’s validation life cycle.3
Contamination risks are challenging because there are two risk subjects that must be included – the product or device, and the patient. The subjects are connected to the source of the contaminant by mechanisms, called systems, that must be clearly identified and structured into risk relationships before the risks can be analyzed.
Contamination risks also have two measures of severity. The first is the level of contamination. The second is the severity of the contaminant’s impact on the patient or risk’s subject. Both severities can range many orders-of-magnitude, up to and including the death of the patient. For effectively managing contamination risks, the analysis team must be careful to appropriately consider and include both subjects and both severity measures.
ReRA is described in detail, with figures and tables, in reference 4. Before continuing, a brief review of ReRA.
Principles Of ReRA
ReRA models all risks as relationships where an initiating event enters and flows through the risk’s mechanism or “system” to produce an outcome event.4 ReRA is based on the following principles:
- ReRA defines a risk as the impact of uncertainty on the actions, activities, mechanisms, processes, and systems that produces an objective or consequence. Because nothing happens spontaneously, all risks are events connected by mechanisms, and it’s the mechanisms that defines the risk. Risks are essentially bad mechanisms that may have an unacceptable likelihood of resulting in a bad outcome.
- Risks are cause-and-effect relationships, where an initiating or cause event of likelihood LC to a mechanism with an expected likelihood of propagating the cause LP to produce an outcome effect of likelihood LE to a subject, where LE = LC * LP. Unless all four elements – cause, mechanism, effect, and subject are identified, the risk has not been sufficiently defined for analysis.
- The risk’s mechanisms must be organized into a System Risk Structure (SRS), defining the complete path of the primary mechanisms from the initiating cause to the outcome to the final subject. The SRS can be subdivided as necessary to elucidate the risk’s mechanisms and intermediate events for analysis.
- The risk’s mechanism is subject to secondary factors including failure mode events that degrades the mechanism’s performance by ΔLP and improvement opportunities that increase the mechanism’s performance for achieving its objective. Secondary factors can be modelled as a risk where the severity of the secondary risk is the change in the primary system’s LP.
- A risk’s outcome or objective has two attributes – severity of impact and uncertainty of occurrence. For effective analysis and communications, both attributes must be defined using a straightforward, universal, quantitative criteria.
- The severity of a risk’s outcome must be defined in terms of its quantitative or expected value assessment of its monetary impact on the subject in terms of value, expense, avoided and remedial costs, etc.
- The uncertainty of an event occurring or a mechanism propagating a cause to produce an outcome is either defined as a probability or a likelihood with a range of zero (impossible) to one (certain).
- A probability is an uncertainty estimate of a risk mechanism or event derived from a statistical analysis of data or calculated using a well-defined mathematical model of the risk’s mechanism.
- A likelihood is an uncertainty estimate of a risk mechanism derived from a subjective analysis of the anticipated performance of the people, equipment, actions, activities, plans, or anything else based on the analysis team’s experience, knowledge, data, or information about the mechanism. If likelihoods are used for estimating a risk mechanism, then the uncertainty of any event it produces is also a likelihood.
- Because of the inherent uncertainty of risks, the likelihood or probability of both risk events and mechanisms can be sufficiently described by a single trial Bernoulli distribution for both probability and likelihood (LX) using an order-of-magnitude “logarithmic-based” integer rating scale LX^ (LX) from ≤ -7 (≤ 0.0000001) to ≥ 7 (≥ 0.9999999) with LX^ = 0 for LX = 0.5. The rating scale is derived in reference 4.
- If the likelihood rating of success for either an event or mechanism is LX, then the likelihood of failure ⌐LX is ⌐LX = 1 – LX and vice versa. For ratings, a corresponding relationship is ⌐LX^ = – LX^.
- The ReRA relationship describes two kinds of risks. A “harm risk” where the risk mechanism’s goal is reducing the likelihood of the harmful outcome to an acceptably low level; and a “benefit risk” where the mechanism’s goal is to maximize the likelihood of achieving the beneficial objective or outcome.
Additional discussion and application of ReRA can be found in references 4, 5, 6, 7, and 8.
Understanding And Modeling Contamination Risks
For controlling contamination, the harm risk approach describes mechanisms or CCSs that have a low likelihood of transmitting or propagating the contaminant while the benefit risk approach describes CCSs that have a high likelihood of protecting the subject from contamination. While the two risk types have the same net effect, low likelihood harm risks behave differently than high likelihood benefit risk mechanisms.
The risk of contamination to a patient is summarized by the two SRS shown in Figure 1.
Figure 1: ReRA’s conceptual system risk structure (SRS) for analyzing and managing contamination risks that includes both risk subjects
The first risk on the left is a contaminant passing through a CCS of likelihood LP to produce a contaminated product or medical device. The second risk is the contaminant from a product or device harming a patient or subject by passing through a sequence of systems with a likelihood of PaLP. The focus of this article is on the first CCS risk required for protecting the product or device.
The second risk is obviously a sequence of many sub-systems or processes that include the product or device’s release mechanisms that might detect the contamination, the decision to administer the therapy or use the device on the patient and, very importantly, the physiological response of the patient to the contaminant. The second risk’s impact and likelihood of occurrence of harm depends on many factors that include the toxicity of the contaminant on the specific patient population. The second risk is outside the scope of this paper. However, the same ReRA principles described for the CCS risk can be applied to an expanded form of the supply chain/patient response risk sequence. The risk model described in Figure 1 is very similar to the model described for medical devices in appendix C of ISO 14971.8
Because the risk as a Contaminant → CCS → Contamination relationship, contamination is the result of an ineffective CCS. The primary purpose of a risk analysis is to support the design of a CCS that has an acceptably low likelihood of the threat resulting in harm by identifying and controlling the important design features of the CCSs’ mechanisms and their failure modes that might increase the likelihood of contaminated product or device eventually reaching the patient or subject.
The CCS may be composed of a sequence of one or more systems that include gowning, cleanrooms, cleaning and disinfection procedures, controlled airflows, etc. The failure modes to the CCS are events or situations that impact the risk mechanism, degrading the probabilistic performance LP of one or more elements of the CCS. The likelihood of many contaminant threats is certain (LT = 1) resulting in the likelihood of harm dependent only on the performance of the CCS (LP = LH). In some cases, the likelihood of the contaminant threat to the CCS is the outcome of a previous risk such as the failure of a container that also can be modeled as an SRS element.
The application of ReRA will be demonstrated by three examples.
Modeling Contamination As A Harm Risk
The most obvious way to analyze contamination risks is as harm risks, where the objective of the mechanism is to reduce the likelihood of contamination to the lowest possible or at least to an acceptable value by a sequence of barriers. The harm risk approach treats risks the same way Reason viewed risks as layers of Swiss cheese.9
The harm risk model can be demonstrated, including estimating and manipulating the likelihood ratings, by the following example of controlling COVID-19 virus exposure.
Example 1 – Controlling a COVID-19 contamination risk
When contamination risks are analyzed as harm risks, the contaminant is separated from the product or risk’s subject by independent barriers in a layers of protection analysis (LOPA).10,11 Figure 2 shows a three-system SRS of a CCS designed to prevent the flow of a COVID-19 virus from a possibly infected person (threat) to the harm of exposing a second person, the subject of the risk.12
Figure 2: SRS of a three-system CCS for preventing COVID-19 exposure.12 The SRS uses likelihood ratings described in the ReRA summary. The three barriers are treated as independent CCS elements. Because all the likelihoods are less than 50%, the overall rating LH^ is the sum of the logarithmic likelihood ratings including the threat rating (0LT^ = - 1) and the three system ratings of ALP^, BLP^, and CLP^. As shown on the SRS and the risk register in Table 1, when all three layers are used properly, the likelihood of contamination is extremely low with 3LH^ = - 7.
If the threat is sick (LT^ = 0) and does not wear a mask (ALP^ = 0), then the likelihood of the subject being exposed is LH^ = - 4 (very low, but possible) if proper distancing is maintained and the subject’s mask is properly used. If physical distance is not maintained and the subject does not wear the N95 mask properly, then the likelihood of infection can become very close to certain or roughly equal to the likelihood the threat is infected.
The risk is further described, including failure modes and improvement opportunities for each CCS element, using the risk register (RR) shown in Table 1.
Table 1: Summary Risk Register for COVID-19 risk’s SRS shown in Figure 2. The risk register uses likelihood ratings described in the ReRA summary.
While the SRS and RR provides an overview of the risk relationship to the subject being exposed, it does not include the risks associated with the subject getting sick.12
In the following example, the same harm ReRA risk model is used to evaluate the risk of product contamination during an aseptic manipulation in a laminar flow hood.
Example 2 – Harm risk model of aseptic manipulations
An important, frequently seen example of contamination risk comes from manipulating a product, such as expanding a cell culture batch, in a laminar flow hood in open containers. The focus of this discussion is on the method used to analyze the risk of airborne contamination. Many details are kept to a minimum or not addressed for brevity in presenting the risk analysis approach.
The aseptic manipulation is summarized in Figure 3 by five systems that separate intermediate risk events on the path of an outside airborne contaminant reaching the product.
Figure 3: SRS harm risk model of an aseptic manipulation in a laminar flow hood. The five possible barriers are like the Swiss cheese model analogy.9 The likelihoods for the events and systems are estimated by a ReRA team using the likelihood rating scale.
The harm risk model in Figure 3 only estimates the risk to the product from outside contamination that could reach the product through the air. The overall risk of a contaminant from outside the cleanroom reaching the product is essentially impossible with an LH^ of - 8 unless impacted by several significant failure modes to several barriers. The SRS shown in Figure 3 is supplemented by the Risk Register shown in Table 2.
Table 2: Risk register for harm risk ReRA model of the aseptic operation described by the SRS in Figure 3. Note that event 2 has been designated as a critical control point (CCP) that could be subject to additional monitoring for potential contamination.
Note that if closed final containers are used, many of the systems may not be necessary to achieve a very low likelihood of contamination.
The RR shows comments and a variety of possible failure modes and improvement opportunities for the risk. The likelihood ratings for each layer are clearly stated and can be used for reaching a consensus by the analysis team or review by regulatory or quality groups. While disagreements in the ratings are to be expected, consensus using the likelihood ratings is possible.
A more complete analysis of the CCS might also include other threats such as the people performing the manipulations. For example, System C also has the threat of contamination by people performing the manipulations. The additional gowning provides a barrier between the contaminants on the operator’s hands from being released into the hood threatening the product through System D.
An underlying assumption of the barrier model is that the performance of each layer is independent of the other layers. In other words, if one layer fails, the other barriers in the sequence are unaffected. Although this assumption is likely valid for many simple contamination risks like the COVID-19 CCS, there may be situations where this approach is inappropriate. In some cases, the performance of a barrier may depend on the performance of the previous layers, much like risks associated with executing a procedure or supply chain.6,7
An alternative to treating each barrier as an independent harm risk is to treat each layer as a benefit risk.
Modeling Contamination As A Benefit Risk
Although somewhat counterintuitive, a more rigorous method of evaluating contamination risks is to model the CCS as a sequence of benefit risks that have a goal of achieving a high likelihood of preventing contamination.
While harm risks are viewed as Swiss cheese like barriers, benefit risks are best viewed as chains where the chain is only as strong as the weakest link. Benefit risk models can be used for modeling procedure and supply chain risks.6,7 A sequence of benefit risks, like a supply chain, requires the success of each link in the chain.
Using the benefit risk approach, each link in the CCS chain must achieve its objective for the CCS to be successful. Experience with regulatory agencies suggests that they frequently view contamination prevention as a sequence of interdependent barriers where the failure of one link raises significant questions about the success of the entire CCS’s barrier sequence.
Using ratings, the overall likelihood rating for a chain with high likelihoods of success (>99%) can be estimated by using a yield approximation, where the lowest likelihood becomes the likelihood rating of the entire sequence.
While the harm model may underestimate the likelihood of a barrier sequence resulting in a contamination event, the benefit model is a far more rigorous approach that may overestimate the likelihood of a contamination because each barrier’s success requires the acceptable performance of the previous barriers. Thus, it may be appropriate to use the harm model for less critical risks and use the barrier model for more significant contamination risks as shown in the following example.
Example 3 – Benefit risk model of aseptic manipulations
Another important threat associated with aseptic manipulations described in example 2 is the threat of the operator’s hands and arms performing the manipulations in the hood. The SRS for the risk of contamination from the operator using gloves is shown in Figure 4. However, the risk will be evaluated as a benefit risk for a more rigorous analysis using a worst-case scenario that the operator’s hands are assumed to be contaminated prior to applying the additional gowning provided by system C.
Figure 4: The SRS shows the certain contaminant threat of the operator’s arms and hands and the path it would follow to the product. The two systems are analyzed as a sequence of benefit risks.
Evaluated as a benefit risk, the overall likelihood of the CCS’s success is the minimum rating of the system sequence. In this case system D with a DLP^ value of 2 (99%) is the limiting risk system. The RR for the benefit risk analysis is shown in Table 3.
Table 3: Risk register for SRS shown in Figure 4, treating systems C & D as benefit risks as barriers against the threat of contamination from the operator.
The benefit analysis predicts a success rate of about 99% (LB^ = 2) or a failure rate of 0.01% (LH^ = - 2) if the failure modes are controlled to the baseline values. With system D being the limiting system, improvement opportunities include detailed procedures to control failure modes that might improve the risk’s success to 99.9% (LB^ = 3).
If the risk shown in Figure 4 is treated as a harm risk, the likelihood ratings can be converted from success ratings to failure ratings using the relationship ¬LX^ = – LX^ to calculate a value of LH^ = - 5. Using both types of risks to evaluate the risk’s SRS can stimulate the discussion among the risk analysis team members to better understand the risk and achieve a consensus.
One form of contamination risks of significant concern in a multiproduct facility is cross contamination between products.
Cross-Contamination Risks
As with normal contamination risks, the risk of cross contamination between products is modeled by building an SRS that describes the complete path that product A would follow to contaminate product B. Cross contamination can occur via several paths. The path for equipment cross contamination is summarized in Figure 5. Similar SRS paths can be developed for other cross-contamination paths.
Figure 5: SRS landscape for cross-contamination threats of product A contaminating product B. The purpose of the risk analysis is to reduce the likelihood of the cross-contamination mechanism sequence to an acceptable likelihood based on the severity of the cross contamination.
Once the path and sequence of systems are identified, baseline XLP for each can be estimated based on the system’s design and their process validation define, design, qualification, and verification activity results. After the baseline SRS’s XLPs are developed, the impact severity and likelihood of occurrence for each failure mode for each SRS system can be identified and evaluated. The risk can be documented by annotating the SRS and completing an RR as shown in the examples.
Contamination Landscapes
Analyzing a jumble of commingled risks usually leads to a great deal of confusion. To adequately analyze and manage a risk, the cause, system (w/failure modes), consequence (w/subject) must be explicitly identified into specific relationships. A complete contamination landscape might include a wide variety of contaminants that might pass through a wide variety of people, material, equipment, airborne, facility, and other possible mechanism pathways to a wide variety of subjects. All these risks must be identified, analyzed, and managed individually as cause-CCS-consequence relationships.
Although some risks may be obvious and easily described and analyzed, there really is no other way for building a complete picture of all the contamination risks. The severity of the contaminant and the nature of the paths will determine whether they merit significant analysis that includes SRS/RR development for each risk.
Discussion & Conclusion
While learning ReRA for modeling risks is initially intimidating, the approach becomes easy once experience teaches that risks are mostly relatively straightforward system paths that might produce bad results rather than just thinking of risks as a tangle of bad outcomes.
An effective risk analysis could allow the use of ALARP principles for aseptic processing concepts where specific risks can be identified to have achieved an acceptable level of control without the use of very expensive extraordinary measures.
The goals of a ReRA are simple. First, to structure the risk, understand the relationship between uncertain events or situations and how they are connected by mechanisms that produce the risk’s outcomes. Once the mechanisms or systems are identified, the likelihood of favorable outcomes can be improved by either modifying the mechanisms, reducing the likelihood of the cause event occurring, if possible, or controlling failure modes to the mechanisms that might decrease the likelihood of success.
One of ReRA’s most important features is its simplicity of the likelihood rating scale by using simple likelihood ratings. The unambiguous rating scale allows the analysis team to reach a clear consensus on the likelihood of the risk’s occurrence. However, the primary purpose of the analysis should always be to identify the failure modes for minimizing the likelihood of failure as well as the identification of improvement opportunities for making the risk less likely to occur.
References
- EU GMP Annex 1, Manufacture of Sterile Medicinal Products, 2023.
- ISO 14971 Medical devices – Application of risk management to medical devices. Third edition 2019-12.
- Witcher, M.F., A Functional History of Process Validation: Part 2 – The Key to a More Effective Future, BioProcess Online, August 24, 2020. https://www.bioprocessonline.com/doc/a-functional-history-of-process-validation-part-the-key-to-a-more-effective-future-0001
- Witcher, M.F., A New Approach for Minimizing Human Errors in Biopharmaceuticals and Medical Devices, Bioprocess Online, February 3, 2025. https://www.bioprocessonline.com/doc/a-new-approach-for-minimizing-human-errors-in-biopharmaceuticals-and-medical-devices-0001
- Witcher, M.F., Relational Risk Analysis for The Bio/Pharma Industry, BioProcess Online, January 29, 2024. https://www.bioprocessonline.com/doc/relational-risk-analysis-for-the-bio-pharma-industry-0001
- Witcher, M.F., Using Relational Risk Analysis to Control Procedure Failures, February 15, 2024. https://www.bioprocessonline.com/doc/using-relational-risk-analysis-to-control-procedure-failures-in-the-bio-pharma-medical-device-industry-0001
- Witcher, M.F., Managing Supply Chain Risks Using Relational Risk Analysis, April 5, 2024. https://www.meddeviceonline.com/doc/managing-supply-chain-risks-using-relational-risk-analysis-0001
- Witcher, M.F., A New Approach to ISO 14971 For Better Medical Device Risk Analysis, Med Device Online, October 23, 2024. https://www.meddeviceonline.com/doc/a-new-approach-to-iso-for-better-medical-device-risk-analysis-0001
- Reason, J., Managing the Risks of Organizational Accidents, Ashgate Publishing, 1997.
- Mains, P. T. Zacharatos, and A McFarland, Assessing Cross Contamination Using Layers of Protection Analysis for Facility and Product Safety, February 14, 2024. https://www.bioprocessonline.com/doc/assessing-cross-contamination-using-layers-of-protection-analysis-for-facility-and-product-safety-0001
- Center for Chemical Process Safety (CCPS), Layers of Protection Analysis – Simplified Process Risk Assessment; American Institute of Chemical Engineers (AIChE), New York, NY, 2001.
- Witcher, M.F., Using System Risk Structures to Evaluate COVID-19 Pandemic Risks” BioProcess Online, December 2021. https://www.bioprocessonline.com/doc/using-system-risk-structures-to-evaluate-covid-pandemic-risks-0001
About The Author:
Mark F. Witcher, Ph.D., has over 35 years of experience in biopharmaceuticals. He currently consults with a few select companies. Previously, he worked for several engineering companies on feasibility and conceptual design studies for advanced biopharmaceutical manufacturing facilities. Witcher was an independent consultant in the biopharmaceutical industry for 15 years on operational issues related to: product and process development, strategic business development, clinical and commercial manufacturing, tech transfer, and facility design. He also taught courses on process validation for ISPE. He was previously the SVP of manufacturing operations for Covance Biotechnology Services, where he was responsible for the design, construction, start-up, and operation of their $50-million contract manufacturing facility. Prior to joining Covance, Witcher was VP of manufacturing at Amgen. You can reach him at witchermf@aol.com or on LinkedIn (linkedin.com/in/mark-witcher).