ML-Powered Medical Devices: 10 Tips For Regulatory Compliance
By Steven F. LeBoeuf, Ph.D., president and co-founder, Valencell
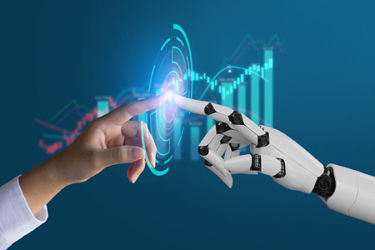
After having survived several pre-sub correspondence letters from the FDA in pursuit of 510(k) clearance for Valencell’s cuffless, calibration-free fingertip blood pressure monitor (BPM) (Not FDA cleared. Not for sale in the U.S. as of this article’s date of publication), I’ve become familiar with the FDA’s Good Machine Learning Practice (GMLP) for Medical Device Development: Guiding Principles,1 which I like for at least two reasons: 1) it’s a strikingly quick read and 2) it’s spot-on. The guidance document outlines 10 guiding principles, each of which is critical in developing medical devices powered by machine learning (ML). In this article, I summarize the 10 principles and provide real-life examples of how you can employ them.
1. Multidisciplinary Expertise Throughout The Product Life Cycle
The key takeaway is that data science cannot work in a vacuum in developing machine learning models for medical devices. It’s critical that a multidisciplinary team of scientists, engineers, clinicians, and regulatory experts is assembled with a deep understanding of the intended use and the clinical problem that is being solved. I emphasize “clinical” here, as data science teams tend to perseverate on optimizing (and in some cases overoptimizing) the machine learning model as opposed to assuring the full system-level clinical solution aligns with the intended use. In addition to building our multidisciplinary team within Valencell, we assigned representatives from each of the key disciplines to meet routinely to align efforts and update timelines as needed.
2. Good Software Engineering And Security Practices
Medtech teams must have good software engineering practices, data quality assurance, data management, and robust cybersecurity practices. At Valencell, our approach to this has been to follow the data from beginning to end in the product development cycle. Doing this helps expose risks and mitigations in terms of maintaining data integrity and security. For example, our product needs to autonomously capture various diagnostics and metadata from the user to be serviced in the field; assuring the safety and integrity of this data became part of our development process.
3. A Focus On The Intended Patient Population
It is critical to ensure the generalizability of the machine learning model across the intended patient population, to confirm the model’s usability, and to identify where the model may underperform. Regulators want assurance that the machine learning model will be accurate across a full range of ages, weights, and heights within the indications for use. This assurance is particularly important for noninvasive medical wearables where metadata is incorporated into the machine learning model to help improve estimation accuracy.
4. Independence Of Training Data Sets And Test Sets
I’ve seen this cardinal rule broken numerous times both in peer-reviewed publications and in FDA-cleared medical devices. Machine learning models always show greater accuracy for data sets used in training than for data sets found in the wild. Since medical devices employing machine learning will ultimately be judged by their performance on subjects never used in model development, subjects used in model training can never be used in clinical validation testing. But training-test independence goes beyond the data sets themselves. It is also important to make sure the machine learning solution has no dependence on the testing environment. For example, in Valencell’s upcoming clinical validation trial, we must collect data from participants in three geographically different locations. The reasoning is that, even with test-train subject independence assured, there is always a risk that training and test data sets are confounded by environmental factors that can bias clinical validation results.
5. Reference Data Sets Based Upon Best Available Methods
The key is to develop your machine learning model with a sufficiently robust reference data set that promotes generalizability across the intended patient population. Don’t make the beginner’s mistake of selecting reference data sets that are just too narrow to ensure model generalizability across the broad patient population (like we did during our early feasibility studies). To achieve the desired accuracy across the entire intended population, you need to boost your reference data set to include a more diverse subject representation.
6. Model Design Aligned With The Intended Use Of The Device
The clinical benefits and risks should be well understood so that clinically meaningful performance goals can be derived while being safe and effective within the intended use. Since blood pressure is not just “one thing” but rather has many meanings depending on the use case, for us it was important to focus on the use case of making blood pressure spot checks easier and more convenient, as opposed to passive monitoring, beat-to-beat monitoring, nocturnal monitoring, and the like.
7. Attention To The Performance Of The Combined Human–AI Team
Humans will always be part of a medical device solution. Even if a physician is not required to interpret the results, at minimum, the patient will be required to interact with the device. The development of the ML solution must consider this factor, particularly with respect to risks and mitigations. Knowing that end users may sometimes misuse the device, it is important to autonomously detect these occurrences and prevent errant measurements where possible. Storyboarding the human-machine interaction is critical in developing adequate mitigations.
8. Demonstration Of Device Performance During Clinically Relevant Conditions
You need to prove the solution is safe and effective for the clinically relevant intended use within the indications for use. Meeting this guideline is more straightforward when clinical relevance has already been established, as with the pursuit of a 510(k).
9. An Ability To Provide Users With Clear, Essential Information
While this bit of guidance may appear demeaningly obvious in developing any medical device, when applied to devices employing ML, special considerations emerge. For example, the ML model may perform differently among different subgroups or within different clinical workflows; this must be effectively communicated to the end users. Consider employing sensor analytics that can determine where differences in model performance are likely, then communicating this to the end user.
10. Monitoring Model Performance Over Time And Managing Retraining Risks
If your product employs a static ML model, retraining/calibration with the user is not required. In contrast, many ML solutions are designed to learn and improve over time – but not without the risk of overfitting, unintended bias, or data drift. These risks need to be managed within the user base as the ML model adapts over time.
Reference
About The Author:
Steven LeBoeuf, Ph.D., is president and co-founder of Valencell. He is an inventor of more than 100 granted patents in the field of wearable biomedical sensing and is an innovator in wearable PPG sensors that are now embedded in millions of wearables on the market today. Before founding Valencell in 2006, LeBoeuf worked on innovations in solid state materials, multiwavelength optoelectronic devices, high-power electronics, nanostructured materials and devices, and biochemical sensor systems while serving as a senior scientist and biosensor project lead for General Electric. LeBoeuf holds a Ph.D. in electrical engineering from North Carolina State University and a BS degree in electrical engineering and mathematics at Louisiana Tech University.