4 Steps To Face The Challenges Of Integrating AI In Your Medtech
By Théophile Mohr Durdez, chief executive officer and cofounder, Volta Medical
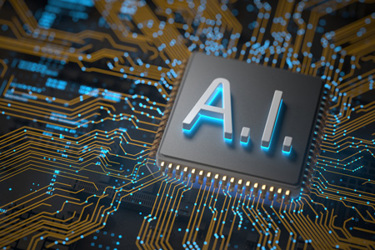
In Season 2, Episode 18 of The Big Bang Theory, the guys try helping Penny expand the market for her hand-crafted barrettes by developing a product line for men. When Penny questions why men would be interested in sparkly, floral hair clips, Howard suggests making the barrettes Bluetooth enabled and Sheldon agrees, noting that “everything is better with Bluetooth.” As silly as that might seem, society seems to have arrived at a similar juncture with respect to its obsession with anything and everything that uses artificial intelligence-based technology. Seemingly overnight, AI has become personal assistant, menu planner, and fitness coach for individuals. The Screen Actors Guild and Screen Writers Guild went on strike this summer, in part, over concerns about the use of AI — instead of human talent — in Hollywood’s creative processes. While the latter ended in September, the former is ongoing. Companies across diverse sectors are using AI-based technologies for enhanced data analytics and knowledge workers are exploring how generative AI tools, such as ChatGPT, can streamline research tasks and development of written materials.
In the medical technology arena, the safe and effective integration of AI into medical devices demands a more deliberate, measured, and purpose-driven approach. Rather than looking for any and every opportunity to deploy AI, device innovators need to identify unmet needs in which AI can be used to analyze past outcomes and incorporate new disease knowledge to predict a clear path toward better health outcomes. Let’s take a look at the four steps to follow.
1. Identify The Setting For AI Enablement To Improve Patient Outcomes
Medical device innovators seeking to deploy AI in meaningful ways need to focus on disease indications and medical procedures in which data analytics can provide improved outcomes compared with what the human mind, eye, and hand can achieve. For example, AI is being used in screening and diagnostic settings to improve detection of polyps and adenomas during colonoscopy and identification of breast tumors in mammography images. In these settings, AI systems enable rates of detection that are superior to what the human eye can achieve, even when that eye resides in the head of an experienced radiologist or gastroenterologist.
While there are many applications of AI in the diagnostic arena, its use in the therapeutic arena has just started emerging. Logically, the first applications of AI in the medical device industry have been developed in highly digitalized specialties and intervention types. For instance, it is being used to improve radiation therapy contouring — the precise mapping of where and how much radiation is delivered to a target area. This increases the safety and efficacy of radiation therapy by enabling delivery of higher radiation doses to the entire tumor while sparing adjacent healthy tissue. On top of this, AI-enabled radiation delivery devices allow radiation to be used in the treatment of additional tumor types and in patients who would not previously have been candidates for treatment.
Cardiac electrophysiology is yet another specialty in which AI enabled systems are being used to improve outcomes for patients undergoing catheter ablation. In traditional ablation procedures, target tissue is identified based on data provided from multiple diagnostic outputs including fluoroscopy, ultrasound, electrical conduction maps, and electrograms (electrical measurements made directly on the heart rather than through the skin). Here again, the development and dissemination of AI technologies are highly facilitated by the huge amount of digital data that is being produced during each cardiac ablation intervention. As of today, extracting the various data generated during a single procedure would require 20 to 50 Gb, depending on the length of the procedure. While physicians are highly trained to interpret electrograms to help guide them in the selection of target tissue, the interpretation increases in complexity with more advanced disease states. AI-based systems can perform calculations more rapidly and have a more comprehensive approach, learning from a greater variety and number of cases than any single electrophysiologist has experienced. In these examples, physicians are overwhelmed by a large amount of data coming from multiple widespread acquisition systems. In both cases, the highly digitalized environment is suitable to AI development and dissemination. As a result, AI improves radiation therapy and cardiac ablation outcomes by aiding physicians in making more informed decisions about which tissue to target.
Importantly, use of these systems can help to reduce the time and associated resources needed for each patient without sacrificing quality by potentially reducing intervention time and reducing the number of disease recurrences. This is an important advantage at a time when health professionals are facing high levels of burn out, hospitals and care centers are facing unprecedented staffing shortages, and there is growing recognition of the need to address entrenched health inequities by making quality care accessible regardless of where a patient is treated.
Medical device innovators seeking to ensure effective deployment of AI to address unmet clinical need should work collaboratively with physicians to develop AI-enabled devices that meet specific clinical needs and eliminate obstacles that simply can’t be overcome using the human brain alone. Obviously, as for other medical device technologies, end users are best suited to identify tasks and procedures for which they need additional support and thereafter to specify the user requirements. But what about analytical tasks that they have learned to perform over the course of their career but are unable to describe? This is where AI may play a role due to its ability to learn from human expertise. As an example, while we can all recognize the faces of our friends, we are often not able to provide a reliable description that would enable someone else to recognize the individual we know so well. In this context, clinicians also can identify procedures in which AI has the potential to enable improved clinical decision-making. The AI-enabled cardiac ablation noted above was developed in collaboration with three electrophysiologists to address their real unmet needs. Designing devices based on user need is the best way to both make meaningful improvements in health outcomes and ensure a market for your new AI-enabled device.
2. Focus On The Training And Testing Data
After identifying a setting in which the use of AI can enable improved outcomes, device innovators need to focus on curating, annotating, and sometimes extracting the machine learning training and testing data that enable optimal device performance. This is why collaboration with expert physicians is essential. The systems in the examples above are trained using hundreds of thousands of data points collected from previously treated patients. The ability of these systems to “learn” is entirely dependent on the quality of the training data. For example, using training data that inaccurately categorizes a mammography finding as malignant when it is fact benign will train your system to make the same mistake. Consequently, training data must be gathered from reliable sources for which there is high confidence that annotations are correct, and the data sets are complete. Device innovators willing to develop AI-enabled systems will need to invest a significant amount of resources in engaging with physicians and designing the tools needed to collect, curate, and annotate data. Making sure that physician experts will be able to dedicate their time to the project despite their overloaded agendas is a critical challenge for success in such collaborations. When curating training or testing data, device innovators also need to be conscious of inherent inequities and biases that may exist within the data and associated annotations and take steps to ensure that the training and validation sets accurately represent the intended population and use for which the device is designed.
3. Address The “Black Box” Concern With Validation Processes
While AI algorithms can be designed for high performance, they also often are viewed as “black boxes” by those outside the realm of data science. This may make it difficult for people to understand — and thus trust — how they work. Robust validation processes can address this concern. Consequently, once an AI-enabled device has been designed and trained, medical technology companies should conduct a rigorous and extensive validation on the bench and/or in a clinical setting.
The validation process should be designed to demonstrate that the levels of safety and efficacy are in accordance with regulatory claims. Whether it is to demonstrate superiority or non-inferiority, this validation process often requires randomized controlled clinical trials to provide robust clinical validation of the novel technology. These trials should be designed to clearly show that the AI-enabled device provides tangible benefit to users and patients. This may be achieved by demonstrating superiority on a clinically relevant endpoint, such as improved diagnostic sensitivity and specificity, reduction in disease symptoms/recurrence, or improved overall/disease-free survival. It may also be achieved by demonstrating that the AI enhanced system can reduce per-procedure or overall healthcare costs, ease patients’ treatment burdens (shorter/fewer treatments), or increase access to care compared with standard approaches.
4. Articulate The Value Proposition To Investors
Finally, companies developing AI-enabled medical technologies must also be able to articulate a business value proposition that resonates with traditional medtech investors (who may be less familiar with AI-based technologies) and traditional tech investors (who may be less familiar with the longer development timelines and evolving regulatory requirements for AI-based medical devices). They must also be able to address the real concerns that patients may have about the use of AI in their medical care as well as the potential use of their own health data to train future medtech innovation. Both audiences deserve clear and transparent communication regarding the potential risks and benefits of both the AI and medtech components of new products and services.
Combining artificial intelligence with human compassion brings together the best that technology and humanity have to offer improving health outcomes. While focusing on the machines, medtech innovators can never lose sight of the parents, children, friends, and co-workers who look to them for hope of a longer and healthier life.
About The Author:
Théophile Mohr Durdez is co-founder and CEO of Volta Medical, a data-inspired electrophysiology company using artificial intelligence, which was founded in 2016 with three cardiac electrophysiologists in Marseille, France. Mohr Durdez and his team work to improve cardiac arrhythmia management by developing data-driven medical devices trained on large databases of procedural data.