The Future Of Medical Device Labeling Is Here
By Gopiganesh Chandra Sekaran, Baxter
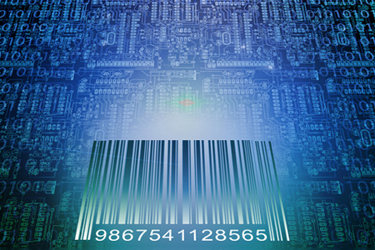
Medical device labeling must show many elements, such as manufacturer information, product information, intended use, indications for use, instructions for use, information regarding sterile conditions, target patient groups, clinical benefits, performance characteristics, residual risks, information on the process for reuse, any restrictions on the combination of devices, human factors, stability, and biocompatibility. Also included are warnings and precautions, which include presence of hazardous substances (e.g., extractables and leachables) with acceptable exposure limits, identification of hazardous materials and associated risks, and any contraindications or limitations to ensure safe use also must be stated.1,2 When a medical device manufacturer places a medical device in any global location, it needs to ensure that it meets the country-specific regulations and language requirements. Labels that lack information or contain inaccurate information may damage the company’s reputation and may lead to product recalls or delays in product release.3,4
Beyond complying with country-specific regulations, many other factors trigger medical device labeling changes. As shown below, there are plenty of reasons why medical device labels can’t be static. They need to be updated to evolve and adapt with all these factors in mind.
Triggers for Labeling Changes:5
- Medical device design modifications
- Change in materials or components
- Software updates
- Changes in intended use/Contraindications
- Changes in global regulation or standards
- Non-conformance labels
- Post-market surveillance data
Developing medical device product labeling involves various functions, including content development, design, implementation, validation, and life cycle management. It requires product owners, SMEs, regulatory, marketing, and manufacturing6 efforts to define technical inputs and participate in content review on an as needed basis throughout the process. Every change requires manufacturers to consistently revise and update labels, coupled with the complexities of compliance with global regulatory requirements, managing translations into multiple languages, and maintaining various formats, such as paper and electronic versions.
Manufacturers must engage in vigilant regulatory monitoring. Centralizing translation processes can streamline operations, ensuring content accuracy and regulatory appropriateness across all markets. In addition, implementing a unified labeling system can facilitate an end-to-end labeling life cycle, which ensures consistency and traceability and reduces potential discrepancies.
Medical device companies spend between 5% and 20% of their total revenue on labeling.7 The amount of spending varies based on the market size and type of device. The higher-risk classification devices (Class III) need to satisfy more complex requirements that may lead to higher labeling costs.
The traditional methodologies rely on people to evaluate every label change with respect to numerous country-specific requirements. This is a time-consuming process, and sometimes costly mistakes may happen due to human error or missing requirements. This further leads to product recalls and damages the company’s reputation in the market.
We already have some smart applications using basic computer programs to manage labeling changes, but they are not smart or flexible enough compared to AI. AI can learn and improve over a period to become a more powerful tool for medical device labeling.
Generative AI For Medical Device Labeling
Generative AI models are trained with large amounts of data, from which they learn the patterns and structures of input and provide us with newly generated data.
Generative AI offers a solution to streamline the labeling development process. During the initial stage we need to select the appropriate AI model and, with the right amount of training data, manufacturers can enhance their labeling practices. We need to provide a distinct range of labeled examples, including product information, device classifications, regulatory requirements, symbols, marketed countries, manufacturer details, economic operator information, and many more. The AI model can learn from sample data to recognize patterns and produce results.
Once we have enough training data, an extensive amount of training is required to fine-tune the AI model for better outcomes. This requires more labeled data, while adjusting its parameters to minimize errors and achieve better accuracy.
Validation is important to ensure that the trained AI model can meet the regulatory requirements and produce reliable results. Multiple levels of testing are needed against our traditional labeling development process to avoid any gaps or discrepancies before deploying the AI model.
Click on image to enlarge.
Automation can help us increase labeling efficiency. For new label development, the artwork and verification steps can be automated. For existing labels, if we need to update the content based on major regulatory changes, we can automate gap identification, redline preparation, label artwork updates, translation, proofreading, and verification. There are numerous possible use cases, and we can customize solutions to meet our needs.
AI can help by identifying gaps and remediating the labels when any new changes impact the existing labels, and it can even create a set of new labels (including unit labels, inner carton labels, shipping cartons, and IFUs) with the appropriate contents. This means labels can be made faster and stakeholder review iterations can be minimized. AI also can make labels easier to read and understand by the intended users (e.g., doctors versus patients). It also can keep track of changes in rules about labeling in different countries. For example, it can send a notification when a new rule is introduced or an existing rule is changed in a country where you sell your devices.
Challenges To Overcome
Even with the promising advancements, there are several obstacles to address when implementing AI in the medical device field. The regulatory frameworks for AI-based medical devices are still in the development stage, and progress toward approval can be complex and time consuming. Regulatory bodies like the FDA require robust evidence of safety and effectiveness, which involves extensive validation studies and clinical trials.8 AI is dynamic in nature, which complicates its regulatory landscape, as continuous changes or updates required to the algorithm may require reevaluation or reapproval by regulatory review bodies. Regulatory bodies may require human validation or intervention to achieve regulatory compliance and accuracy in output.
Another critical concern is the possibility that AI could produce biased results. A system may exhibit biased decisions or output based on the historical data used to train it. Sometimes the data provided may not be sufficient for a new scenario. These situations may lead to discrepancies in labels that might compromise patient safety. So, it is essential that AI systems are ethically designed and implemented by the subject matter experts.
Although AI has potential, human oversight is still needed. Regulators require human validation to ensure safety and effectiveness. So, balancing the benefits of AI with the need for human intervention is one of the key challenges.
In addition, AI integration in medical device labeling faces concerns about how reliable these systems are and the possibility for reduced human intervention. Regulatory bodies may want to understand how the AI reached its conclusion, its decision-making process, and its reliability for providing error-free labeling content. This requires transparency in AI algorithms.9 However, many AI systems are complex and described as “black boxes,” making it difficult to explain their decision-making process. AI systems must provide explainable and interpretable results to ensure that regulatory bodies can understand and validate the recommendations made by these systems, thus ensuring that AI serves as a supportive tool rather than a replacement for human judgment.
Conclusion
There is huge potential for using AI in medical device labeling, streamlining the overall processes and enhancing the accuracy and presentation of clear information to healthcare professionals and patients. We can automate and optimize the creation of medical device labels while ensuring regulations are met and reducing rework. Using natural language processing (NLP) and machine learning algorithms, AI can analyze vast amounts of regulatory information, clinical data, and regulatory body feedback to generate precise labeling content. The automation approach not only saves time and resources but also ensures that labels are consistently updated and aligned to reflect the latest safety information and usage guidelines.
In addition, AI will enhance the readability and usability of medical device labels. It will give us more flexibility to personalize the labeling content based on the end user’s literacy level. The AI algorithms can simplify complex medical terms and translate them into simpler and more understandable language. This will improve patient adherence to device usage instructions and reduce the likelihood of misuse. Interactive applications powered by AI chatbots can further engage users by providing customized information through digital platforms that enhance the experience.
Overall, the integration of AI in medical device labeling represents a significant advancement by improving patient safety and regulatory compliance. AI-driven labeling solutions can solve many challenges related to manual label creation, including ensuring accurate, consistent, and timely completion of labeling updates. It ultimately enhances patient understanding and brings better health outcomes. As the industry continues to evolve with more regulatory requirements, using AI in the labeling process will be unavoidable and may lead to more innovation and efficiency in regulatory affairs and patient care.
References
- https://www.fda.gov/medical-devices/device-labeling/general-device-labeling-requirements
- https://eur-lex.europa.eu/legal-content/EN/TXT/?uri=CELEX%3A32017R0745
- https://www.fda.gov/medical-devices/postmarket-requirements-devices/recalls-corrections-and-removals-devices
- http://www.imdrf.org/docs/imdrf/final/technical/imdrf-tech-190325-wg-labeling.pdf
- https://www.fda.gov/regulatory-information/search-fda-guidance-documents/deciding-when-notify-fda-labeling-changes
- http://www.imdrf.org/docs/imdrf/final/technical/imdrf-tech-190325-wg-labeling.pdf
- https://guidingmetrics.com/content/medical-device-industrys-9-most-critical-metrics/
- https://www.fda.gov/medical-devices/software-medical-device-samd/artificial-intelligence-and-machine-learning-aiml-enabled-medical-devices
- https://www.fda.gov/files/medical%20devices/published/US-FDA-Artificial-Intelligence-and-Machine-Learning-Discussion-Paper.pdf
About The Author:
Gopiganesh Chandra Sekaran is a senior project manager with over 18 years of experience in product design and regulatory compliance within the medical device industry. He has made notable contributions to the European Union Medical Device Regulation (EU MDR) labeling program and has successfully led compliance initiatives across various domains, including FDA’s Unique Device Identification (FDA-UDI), ISO Luer Standards, test method validation, xUDID, and non-conformance reports/corrective and preventive actions. He holds a Bachelor of Technology (B.Tech.) degree in polymer technology from Anna University, Chennai, India. For further inquiries, he can be reached at gopiganeshc@gmail.com.