Adaptive AI-Driven Medical Devices In The U.S.: Ethical Considerations
By Jessica Chen and Thuha Tran, San Jose University
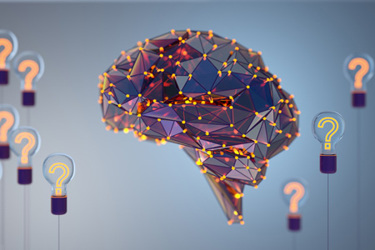
Adaptive AI heavily utilizes large language models (LLM) to imitate human rationality and communication, and sometimes in a more efficient way. In the United States, the FDA has addressed the recent boom in AI technologies. In our first article, we discussed FDA regulatory considerations surrounding these technologies.
There are also ethical concerns regarding patient health data, safety, and privacy, which adds an additional layer of complexity to the design and development of these devices. We focus this article on these concerns. Among the top concerns are exaggerating research bias and posing a danger to patient safety and privacy.
Risk Of Exaggerating Research Bias
Adaptive AI systems carry the inherent risk of amplifying research biases. If the language model is trained on data that inadequately represents underrepresented groups, it may lead to inferential errors, perpetuating and reinforcing existing stereotypes.
Artem Trotsyuk, Ph.D., an AI Ethics and Policy Fellow at Stanford University School of Medicine, specializes in evaluating the unintended consequences of AI in biomedicine and developing strategies to mitigate them. According to Trotsyuk, this potential issue can be effectively addressed by actively involving communities and stakeholders in the AI research and implementation process. This can integrate the values, needs, and concerns of those that are directly affected by the technology, effectively creating a more equitable tool that serves a wider range of concerns. By doing so, we can also ensure a more representative and fair approach to AI development, minimizing the risk of reinforcing biases and promoting equitable outcomes in healthcare.1
The Potential Decrease In Physician Autonomy
Joseph Carvalko, BSEE, JD, who chairs the Technology and Ethics Working Research Group at Yale’s Interdisciplinary Center for Bioethics, raises an important concern about the evolving role of AI in healthcare. He suggests that “much of a doctor's autonomy or decision-making responsibilities may be transferred to AI systems.”2 In this scenario, doctors could potentially find themselves legally accountable for overriding a machine's decision based on the latest technological advancements. This raises a critical ethical question: Should humans be held accountable for the decisions made by AI systems? This notion raises a multitude of complex ethical, legal, and societal debates about responsibility in a world where AI plays an increasingly larger role in decision-making. What was once a clear human responsibility is now entangled with AI, leading to uncertainty about who or what should be held accountable. As AI continues to play a more significant role in healthcare decision-making, addressing this question becomes crucial in ensuring accountability, transparency, and ethical integrity in the medical field.2
Risk To Patient Confidentiality
Personal identifiable information (PII) poses a significant privacy concern when it can be inferred from patient data, such as through ZIP codes. This information can potentially compromise patient confidentiality and raise ethical concerns about data privacy and security. To address these issues, it is essential to adhere to “strict norms and standards including strong multifactor authentication, access control through a neatly designed approval matrix, encryption of sensitive data and transmission.”3
Furthermore, ensuring that the correlational structures in data sets accurately reflect the actual population data is crucial for making precise predictions and inferences. Protecting patient health data has always been a key concern in the healthcare sector, but with the integration of advanced technology — especially in medical device organizations handling large volumes of sensitive data — it has become even more crucial. By implementing these measures, medical device organizations can enhance data privacy, protect patient confidentiality, and maintain the trust and confidence of patients and stakeholders.
The Potential For AI Systems Containing Clinical Data To Be Hacked
The usage of AI technology in the medical landscape is not just utilized to the development of improved clinical data managing systems but also goes hand-in-hand with the storage of confidential clinical data. With the storage of such data in a centralized location, the need for a sound infrastructure that addresses the safety of keeping such information encrypted is essential.3 To address these challenges proactively, it is essential to implement a reactive framework that can swiftly respond to emerging issues while also balancing access limitations to prevent misuse. Among various recommendations, practices such as measuring and mitigating data bias can help ensure fair and equitable AI outcomes. Additionally, incorporating design-specific features, such as face blurring in ambient intelligence systems, can enhance privacy protections and prevent unauthorized data access. By adopting these proactive measures, we can better manage and safeguard the data collected, reducing the risk of misuse and maintaining the integrity and trustworthiness of AI technologies.
Conclusion
With the significant breakthrough of adaptive AI, there is a shared responsibility among all stakeholders to ensure that this transformative technology is used responsibly and ethically. Adaptive AI has the potential to revolutionize various industries and improve countless aspects of our lives. In an attempt to harness these potentials, regulatory bodies like the FDA have proposed regulatory frameworks to govern AI applications in medical products. In 2019, the FDA focused on a Total Product Lifecycle approach for AI/machine learning-based SaMD, highlighting the importance of ensuring the safety and efficacy of these innovations. However, with great power comes great responsibility.
Developers, researchers, policymakers, and users alike must collaborate and establish clear guidelines, ethical standards, and governance frameworks to prevent potential misuse, safeguard data privacy, and promote equitable access and benefits. They must also recognize their role in ensuring the ethical development and deployment of adaptive AI, allowing it to be implemented safely and responsibly. Researchers and thought leaders have raised concerns about potential gaps in research bias, patient privacy, and due diligence at the research and governmental levels. Within the research community, AI systems must include built-in safeguards, such as restrictive loops in data gathering, aiming to mitigate biases and ensure ethical use.
As with any breakthrough technology, the immense power of adaptive AI comes with profound accountability. By fostering collaboration among all stakeholders—developers, researchers, regulators, and users alike—we can harness the full potential of adaptive AI while minimizing risks, protecting patient privacy, and ensuring its responsible and beneficial integration into society. Through proactive efforts, we can promote responsible innovation and allow adaptive AI to enhance our lives and the public sector safely and ethically.
References
- https://www.linkedin.com/pulse/mitigating-unintended-consequences-ai-biomedicine-colangelo-xxyhf/
- https://medicine.yale.edu/news/yale-medicine-magazine/article/hard-choices-ai-in-healthcare/
- https://ihf-fih.org/news-insights/artificial-intelligence-and-cybersecurity-in-healthcare/
About The Authors:
Jessica Chen is a recent Medical Product Development Management master’s of science graduate at San Jose State University. She holds a background in nursing, with a B.S. from the Valley Foundation School of Nursing at San Jose State University. Her interests lie within the medical device industry, particularly in the post-market surveillance sector, focusing on medical device vigilance, pharmacovigilance, and adverse event reporting. Chen has particular interest in improving patient safety and ensuring the effectiveness of medical devices through rigorous monitoring and reporting processes.
Thuha Tran has earned a master's degree in medical product development management at San Jose State University and holds a bachelor's degree in microbiology. She has experience in next-generation sequencing and project management for regulated medical devices. Highlights in her experience include supporting high-throughput sequencing pipelines, assisting in sensitive R&D experiments extracting and pooling DNA from live cells, and supporting the PMA approval for a cancer screening product. She currently works in project management for FDA- and EUMDR-regulated electrophysiology products.