Medtech Data Analysis: Techniques to Create Business-Building Insights
By Bill Woywod and Catherine Ellis, Guidehouse
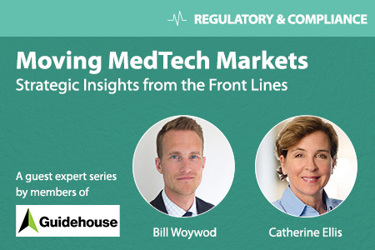
A medical technology company looked to acquire a new technology that seemed like a perfect fit for its existing call points. However, a rigorous claims analysis revealed the clinical specialists called on with its existing portfolio only treated 14 percent of indicated patients, and a different specialty with whom it had no prior experience treated nearly 60 percent of patients.
The due diligence analysis now had to incorporate an unanticipated set of investments – driving indicated patients to its current customers, training its customers to use the technology, and building in sales capacity for a new set of customers.
This is just one example of how harnessing rich data can help medtechs go to market smarter. Today, masses of facts and statistics — from repositories of public and commercial claims to electronic medical records, sales and account statistics, pricing databases, local epidemiology information, and more — threaten to overwhelm marketers interested in mining them to unveil critical market insights. Combining industry expertise with sophisticated data science methodologies offers medtechs a more precise and detailed understanding of market dynamics, enabling better business decision-making.
To this end, medtechs of all sizes are increasingly tapping into machine learning and quantitative longitudinal claims analysis to gain competitive advantages.
Machine Learning Fine Tunes Segmentation and Targeting
Machine learning produces consistent and sometimes dramatic improvements over traditional approaches to targeting and segmentation. In machine learning, a computer sifts through data set samples (with no size limitations) and “learns” to distinguish and sort the records, eventually iterating millions of combinations of variables to drill down into specific linkages.
This process allows the computer to identify the most predictive variables from all data to make predictions and perform classifications based on that information, while minimizing the opportunity for human bias. Furthermore, as new data is available and integrated, machine learning enables an iterative process that perpetually improves its predictive power throughout a product lifecycle.
With the benefit of machine learning, decision-makers can answer questions like:
- My product uptake is leveling off — where are the next best providers and/or patients?
- How do customer sets behave differently, and how should we approach each?
- What treatment pathways are our ideal patients likely to travel, and where can we most effectively interject?
- Is our field team right-sized, or do we need to resize and realign representatives?
- For our technology, what is an accurate, achievable forecast?
For example, a small biotech with a rare disease gene therapy was struggling to identify likely users — even many specialists were unfamiliar with the rare indication. Leveraging an extensive database including physician claims history and field team input via CRM extracts, a machine learning model was trained to identify highly specialized physicians with knowledge of the rare disease.
The final model identified an additional 1,600 high-disease knowledge physicians, a significant increase compared to the 220 the field team initially called on.
Longitudinal Claims Analysis Refines Sizing and Utilization Rate Estimates
Quantitative longitudinal claims analysis is another effective methodology that could benefit medtechs seeking a competitive advantage. Used skillfully, it uses a standardized and validated process to synthesize commercial and public sources of claims data, producing precise all-payer estimates.
These estimates can be programmed to account for variation in age distribution across payer types, as well as denied claims and claims adjustments, to provide detailed, accurate, and actionable insights. Companies use this methodology to pinpoint the patients, settings, specialists, and payers that could benefit most from their technologies.
In a recent case, longitudinal claims analysis helped a company understand why one of its core devices was experiencing declining sales. Originally designed for in-office procedures where reimbursement generously rewarded practitioners, procedures had gradually shifted to ambulatory surgery centers (ASC) and hospital outpatient departments (HOPD), where reimbursement was tighter, and scheduling more complex and time-consuming for both practitioners and patients.
The shift mapped neatly with the decline in sales. Further inquiry revealed that clinicians were unsure how to administer local anaesthesia and uncomfortable with the possibility of patients in pain. Therefore, they shifted to the general anaesthesia available in ASC and HOPDs. However, the inconvenience and lower reimbursement of ASCs and HOPDs inadvertently encouraged both clinicians and patients to explore other therapeutic options.
Armed with this insight, the company shifted investment from sales to training, teaching clinicians to administer local anaesthesia correctly so they’d have confidence to conduct the procedures in an office setting.
In another example, a company leveraged provider-level data to gain a richer understanding of its customers. Before conducting the assessment, company leadership believed most patients were under the care of specialists. However, detailed analysis indicated that various mid-level practitioners (e.g., nurse practitioners) managed the majority of patients. As a result of this insight, the company adjusted its field strategy to more appropriately target those practitioners, and in so doing was able to more than double its coverage.
Longitudinal Claims Analysis Pinpoints Patients and Pathways
Claims-based patient analysis can also provide insight into clinical practice care pathways. Multiyear medical claims from Medicare and commercial insurers can be used to track patients longitudinally to assess care over time. Knowing the care pathway is unique to each patient, organizing these pathways according to macro-level similarities for a single condition can characterize the interaction among patients, providers, and payers in real-time.
Furthermore, the detail available in claims data is extremely rich, including millions of patients, as well as information regarding diagnosis (Dx), prognosis (Px), and other specialty codes. This makes it possible to precisely quantify a variety of discrete patient pathways to inform key business, market development, and commercialization decisions.
For example, the leadership team of an orthopaedic medtech with a long and complex patient care pathway sought to identify the optimal point to insert its technology. The clinical view — offered by clinicians based on their understanding of optimal patient care — presented a linear, logical flow through the system as patients progress from conservative care to more aggressive therapy.
However, when a longitudinal model was constructed using claims data from millions of patients, it indicated a minority of patients pass through the system linearly. In fact, most patients bounce from provider to provider in an uncoordinated, chaotic fashion. But the longitudinal analysis was able to identify two or three key common patient touchpoints amid the chaos. The company was then able to use these as key anchor points to identify, “catch,” and refer patients to receive the therapy.
High-Powered Data Science Drives Better Strategies
In these and many other real-life examples, the insights gleaned from sophisticated claims data analysis methodologies helped medtech companies avert significant risks and more acutely align their business strategies to the realizable market potential of their technologies. As market shares grow ever more difficult to seize, leadership teams that tap into high-powered, high-capacity data science capabilities are more likely to find and capitalize on the “needles” of insight buried in the ever-growing haystacks of data.
Additional Resources
About the Authors
Catherine Ellis is a director in Life Sciences practice of Guidehouse primarily assisting medical device and technology clients in both developed and emerging markets. She works with a variety of clients of different sizes – from global corporations to smaller, single-technology companies. With expertise in both acute and post-acute settings, she collaborates with clients to assess growth opportunities, develop business and market-access strategies, and provide commercial support, including due diligence, market assessment and development, forecasting, portfolio analysis, and competitive intelligence.
Bill Woywod, MPH, MBA, is a managing consultant in the Life Sciences practice at Guidehouse, where he leads big data analytics and machine learning projects. He has played a central role in expanding Guidehouse’s data analytics capabilities, including the development and execution of sophisticated claims data analyses and machine learning initiatives for clients in the medtech and biopharma industries. Previously, Bill worked as a data scientist at a large regional health plan, where he led the development and successful execution of the organization’s first machine learning program.